Virtual Screening of Phytochemicals to Novel Target (HAT) Rtt109 in Pneumocystis Jirovecii using Bioinformatics Tools
Ramya Sugumar1, Abhinand Ponneri Adithavarman2, Anusha Dakshinamoorthi3, Darling Chellathai David4, Padmavathi Kannan Ragunath5
1 Assistant Professor, Department of Pharmacology, Sri Ramachandra University, Tamil Nadu, India.
2 Ph.D Research Scholar, Deapartment of Bioinformatics, Sri Ramachandra University, Tamil Nadu, India.
3 Associate Professor, Department of Pharmacology, Sri Ramachandra University, Tamil Nadu, India.
4 Professor and Head, Department of Pharmacology, Sri Ramachandra University, Tamil Nadu, India.
5 Professor and Head, Department of Bioinformatics, Sri Ramachandra University, Tamil Nadu, India.
NAME, ADDRESS, E-MAIL ID OF THE CORRESPONDING AUTHOR: Dr. Ramya Sugumar, 22/13 3rd Street V.O.C Colony, Aminjikarai, Chennai, Tamil Nadu-600029, India.
E-mail: drramya.sugumar@gmail.com
Introduction
Pneumocystis jirovecii is a fungus that causes Pneumocystis pneumonia in HIV and other immunosuppressed patients. Treatment of Pneumocystis pneumonia with the currently available antifungals is challenging and associated with considerable adverse effects. There is a need to develop drugs against novel targets with minimal human toxicities. Histone Acetyl Transferase (HAT) Rtt109 is a potential therapeutic target in Pneumocystis jirovecii species. HAT is linked to transcription and is required to acetylate conserved lysine residues on histone proteins by transferring an acetyl group from acetyl CoA to form e-N-acetyl lysine. Therefore, inhibitors of HAT can be useful therapeutic options in Pneumocystis pneumonia.
Aim
To screen phytochemicals against (HAT) Rtt109 using bioinformatics tool.
Materials and Methods
The tertiary structure of Pneumocystis jirovecii (HAT) Rtt109 was modeled by Homology Modeling. The ideal template for modeling was obtained by performing Psi BLAST of the protein sequence. Rtt109-AcCoA/Vps75 protein from Saccharomyces cerevisiae (PDB structure 3Q35) was chosen as the template. The target protein was modeled using Swiss Modeler and validated using Ramachandran plot and Errat 2. Comprehensive text mining was performed to identify phytochemical compounds with antipneumonia and fungicidal properties and these compounds were filtered based on Lipinski’s Rule of 5. The chosen compounds were subjected to virtual screening against the target protein (HAT) Rtt109 using Molegro Virtual Docker 4.5. Osiris Property Explorer and Open Tox Server were used to predict ADME-T properties of the chosen phytochemicals.
Results
Tertiary structure model of HAT Rtt 109 had a ProSA score of -6.57 and Errat 2 score of 87.34. Structure validation analysis by Ramachandran plot for the model revealed 97% of amino acids were in the favoured region. Of all the phytochemicals subjected to virtual screening against the target protein (HAT) Rtt109, baicalin exhibited highest binding affinity towards the target protein as indicated by the Molegro score of 130.68 and formed 16 H-bonds. The ADME-T property prediction revealed that baicalin was non-mutagenic, non-tumorigenic and had a drug likeness score of 0.87.
Conclusion
Baicalin has good binding with Rtt 109 in Pneumocystis jirovecii and can be considered as a novel and valuable treatment option for Pneumocystis pneumonia patients after subjecting it to invivo and invitro studies.
Introduction
Pneumocystis jirovecii is an opportunistic fungal pulmonary pathogen which causes pneumonia in HIV or other immunosuppressed patients [1]. Pneumocystis pneumonia is an important cause of mortality and morbidity, with mortality rates ranging between 10-30% in HIV infected patients and 30-70% among other immunosuppressed patients [2–7].
The pharmacological treatment for Pneumocystis pneumonia includes trimethoprim-sulphamethoxazole, atovaquone, clindamycin, pentamidine, trimetrexate plus leucovorin and prednisone as an adjunctive agent. Among them the drug of choice is trimethoprim-sulphamethoxazole which acts by inhibiting folic acid synthesis [1].
With these currently available non-specific therapeutic agents, the treatment of Pneumocystis pneumonia is challenging due to development of resistance among Pneumocystis jirovecii and considerable adverse effects associated with these drugs. Hence, there is a need to develop drugs against novel targets with minimal human toxicities [8–13].
Histone acetyl transferase (HAT) Rtt109 is a potential therapeutic target in Pneumocystis jirovecii species. HAT is required to acetylate conserved lysine residues on histone proteins by transferring an acetyl group from acetyl CoA to form e-N-acetyl lysine. HAT mediated acetylation permits transcriptional access to DNA by neutralizing the positive histone charge. Thus inhibitors of fungal (HAT) Rtt109 may be valuable and novel therapeutic agents in Pneumocystis pneumonia [14].
In this study the screening of phytochemicals against (HAT) Rtt109 using in-silico drug designing approach has been carried out.
One of the widely used modern techniques in drug discovery is virtual screening or in silico biological screening. It is a computational technique used to search libraries of small molecules with an intention to identify those structures which are most likely to bind to a drug target (protein receptor or enzyme) [15,16]. There are two broad screening techniques – ligand based and structure based.
Ligand based technique involves building a model of the target by exploring information contained in a given set of structurally diverse ligands that binds to it. These are known as pharmacophore models. A candidate ligand can then be compared to the pharmacophore model to determine whether it is compatible with it and therefore likely to bind [17].
Structure based virtual screening involves docking of candidate ligands into a protein target followed by applying a scoring function to estimate the likelihood that the ligand will bind to the protein with high affinity [18].
Aim
In this study the screening of phytochemicals against (HAT) Rtt 109 using virtual screening/in silico drug designing approach has been carried out.
Materials and Methods
Flow chart illustrating the target identification, modeling & validation and lead identification & validation.

The entire proteome of Pneumocystis jirovecii (3402 proteins) was accessed from Uniport Proteome Knowledge Base. A total of 364 proteins were found to be associated with house-keeping. The ideal protein target was identified using subtractive proteomic approach by assessing the extent of sequence similarity shared by Pneumocystis jirovecii house keeping proteins against the human proteome. Following alignment with Homo sapiens proteome, only those proteins whose similarity was extremely low (<10%) were selected. Histone acetyl transferase (HAT) Rtt109 was selected as an ideal therapeutic target as no sequence homologies were found in humans [13].
Homology Modeling and Validation of Target Protein [
Table/Fig-1]
A 380 amino acid long (HAT) Rtt109 sequence was retrieved from Uniport. Owing to the absence of an experimentally substantiated 3D structure, (HAT) Rtt109 was modeled using Homology modeling (Comparison method). Using Swiss Modeler and i-Tasser for identifying the ideal template, a position specific iterated blast was performed against the protein data bank database. Following which, a suitable template which shares a high degree of sequence similarity with Pneumocystis jirovecii (HAT) Rtt109 was identified.
The tertiary structure of Pneumocystis jirovecii (HAT) Rtt109 was modeled by Homology Modeling by using the chosen template. The structure and functions of the model were then validated using Ramachandran plot and Errat 2 [19,20].
Comprehensive text mining was performed to identify phytochemical compounds with antipneumonia and fungicidal properties. These compounds were filtered based on Lipinski’s Rule of 5. The chosen compounds were subjected to virtual screening against the target protein (HAT) Rtt109 using Molegro Virtual Docker 4.5. Finally, Osiris Property Explorer and Open Tox Server were used to predict ADME-T properties of the chosen phytochemicals [21].
Results
Homology Modeling and Validation of Target Protein
After performing Psi BLAST of the target protein sequence, Rtt109-AcCoA/Vps75 protein from Saccharomyces cerevisiae (PDB structure 3Q35) was chosen as the template with Query coverage of 70% and an identity of 42% with a low E value of 4×10-23. Target protein (HAT) Rtt109 modeled using Swiss Modeler, with Rtt109-AcCoA/Vps75 as a template, was chosen with Pro SA score of -6.57 and Errat2 score of 87.34 [Table/Fig-2a]. Validation analysis by Ramachandran plot for the model revealed 97% of amino acids were in the favoured region [Table/Fig-2b].
(a) Structure of modeled protein target (HAT) Rtt109 depicted in secondary structure cartoon style. Beta pleats are depicted in cyan blue and alpha helices in red. (b) Steric validation of model by Ramachandran plot showing 97% of amino acids in the favoured region.

Lead Identification and Validation
The following phytochemical compounds with antipneumonia, fungicidal properties and following Lipinski’s Rule of 5 were chosen: Ascorbic acid, berberastine, berberine, cinnamaldehyde, diallyltrisulfide, bilobalide and baicalin. When subjected to virtual screening against the target protein (HAT) Rtt109 using Molegro Virtual Docker 4.5, all the compounds interacted with target except Bilobalide and diallyltrisulfide. The Molegro score and number of H bonds formed for Baicalin was -130.682 and 16 bonds, Berberastine -130.223 and 6 bonds, Berberine -129.114 and 7 bonds respectively. The result of virtual screening has been depicted in [Table/Fig-3].
Results of virtual screening of phytochemicals against target using Molegro Virtual Docker 4.5
Phytochemical compound | Molegro Score | Rerank score | H-Bond | Interacting Amino-acids |
---|
Ascorbic acid | -86.1608 | -82.1541 | -18.684 | Val80, Thr81, Ala83, Phe125, Arg127, Tyr132, Trp155 |
Baicalin | -130.682 | -71.4504 | -16.3636 | Asp84, Ser85, Gly87, Ser128, Pro130, His142, Lys143, His144, Ile145, Leu146 |
Berberastine | -130.223 | -99.3681 | -6.98406 | Tyr63, Ser85, Arg127, Gln129, Trp155 |
Berberine | -129.114 | -83.9257 | -7.70195 | Tyr63, Ser85, His144, Trp155 |
Cinnamaldehyde | -79.3204 | -67.1018 | -2.5 | Ala83, Thr102 |
*Diallyltrisulfide, bilobalide failed to interact with target.
Toxicity Prediction of Phytochemical Compounds
Osiris Property Explorer and Open Tox Server used to predict ADME-T properties of the chosen phytochemicals revealed cLogP value and drug likliness score of -1.33 and 0.84 for baicalin, -2.46 and 0.02 for ascorbic acid. All compounds were non mutagenic and non carcinogenic except cinnamaldehyde. The ADMET properties of phytochemicals are described in [Table/Fig-4].
ADME-T properties of phytochemical compounds.
Phytochemical Compound | cLogP | Solubility L/mol | TPSA (A°)2 | Drug likeness | Drug score | Molecular weightgms | Mutagenic | Tumourigenic | Reproductive effect |
---|
Baicalin | -1.33 | -2.72 | 183.2 | 0.84 | 0.67 | 446 | No risk | No risk | No risk |
Ascorbic acid | -2.46 | -0.35 | 107.2 | 0.02 | 0.16 | 176 | No risk | No risk | No risk |
Berberine | 0.52 | -4.67 | 40.8 | -0.67 | 0.49 | 336.0 | No risk | No risk | No risk |
Cinnamaldehyde | 1.61 | -2.23 | 17.07 | -6.47 | 0.18 | 132.0 | Medium risk | High risk | No risk |
Discussion
Pneumocystis pneumonia caused by Pneumocystis jirovecii is an important cause of mortality and morbidity among HIV infected and other immunosuppressed patients [1]. As the conventional antifungal agents are associated with drawbacks such as development of resistance and considerable side effects it has necessitated the need to develop novel drugs devoid of these complications [22].
In order to develop novel drugs, i.e transcription inhibitors for the treatment of Pneumocystis pneumonia, (HAT) Rtt109 was chosen as the target that are widely conserved in fungi but absent in humans [14].
Homology modeling used to model the target (HAT) Rtt109, refers to constructing an atomic-resolution model of the "target" protein from its amino acid sequence and an experimental three-dimensional structure of a related homologous protein (the "template") [23]. Homology modeling was carried out using Swiss Modeler which refers to a structural bioinformatics web-server for modeling 3D protein structures and I-TASSER (Iterative Threading Assemmbly Refinement) which is a bioinformatics method for predicting three-dimensional structure model of protein molecules from amino acid sequences [24]
Rtt109-AcCoA/Vps75 protein from Saccharomyces cerevisiae (PDB structure 3Q35) was chosen as the template as it had a low E value (4×10-23). The expect value (E) is a parameter that describes the number of hits one can “expect” to see by chance when searching a database of particular size. The lower the E value (closer to zero) the more significant the match is and thus justifying the appropriate choice of template [25]. The Model constructed with Swiss Modeler with ProSA score of -6.57 was chosen indicating that it had least errors in 3D structure of proteins. Further this model had an Errat 2 score of 87.34 suggesting that the model was relatively error free and reliable as Errat 2 analyzes the statistics of non-bonded interactions between different atom types [26].
Molegro Virtual Docker was used to virtually screen the chosen phytochemicals against the modelled target in order to predict their protein ligand interactions [27]. Among all the phytochemical compounds Baicalin exhibited highest binding affinity towards the target protein as indicated by the Molegro score of 130.68 and formed good number of H-bonds (16 in number) [Table/Fig-5a&b].
(a) Position of Baicalin within the cavity of the modeled (HAT) Rtt109. (b) Baicalin interaction with target and positions of amino acids involved in the formation of H bonds (asp84, ser85, gly87, ser128, pro130, his142, lys143, his144, ile145, leu146).
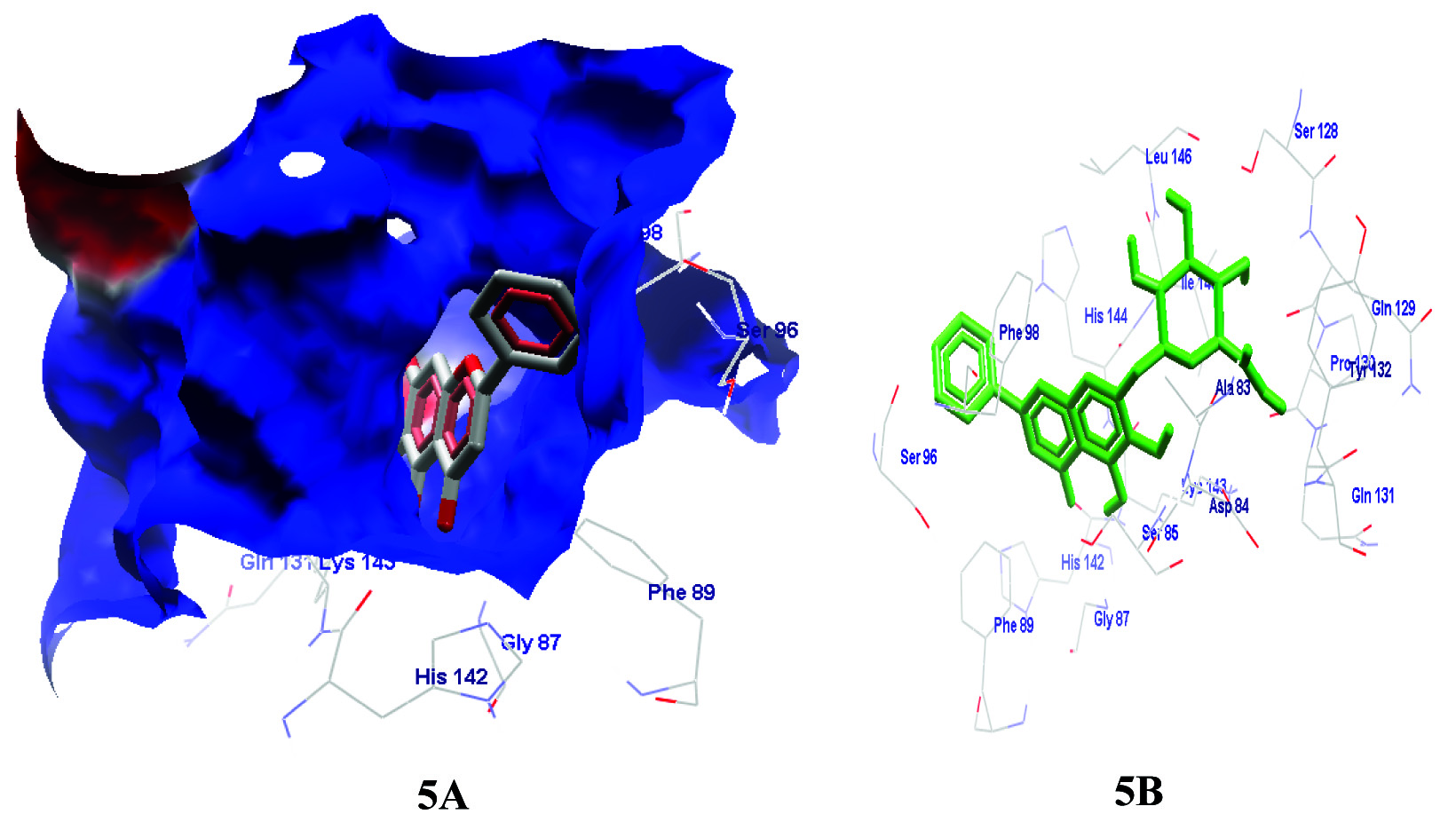
Among all the phytochemicals the ADME-T property prediction of baicalin was the most promising as it was non-mutagenic, non-tumorigenic and had a clog p-value of -1.33 and drug likeness score of 0.87. A low clog p-value indicates that the compound has high hydrophylicity and better absorption. The compound also has a high drug likeness score indicating that it is a potential drug candidate [28].
Baicalin a flavone, is mainly obtained from the root of Chinese medicinal herb Huang-chin (Scutellaria baicalensis). Studies have shown that Baicalin induces apoptosis in pancreatic cancer cells [29]. It is also known to reduce TNF-alpha and sICAM-1 levels, and relieves lung inflammation in rats infected with Pneumocystis carinii [30]. Recently another study has demonstrated that Baicalein a metabolite of baicalin attenuates the quorum sensing-controlled virulence factors of Pseudomonas aeruginosa and relieves the inflammatory response in P. aeruginosa-infected macrophages by downregulating the MAPK and NFκB signal-transduction pathways [31]. Hence, in addition to this anti-inflammatory action, the contributory transcription inhibitory action of baicalin can be helpful in the efficient management of Pneumocystis pneumonia infection.
Limitations
Lack of validation of results with in-vivo and in-vitro studies.
Conclusion
This in-silico study suggests that Baicalin has efficient binding affinity with (HAT) Rtt109 in Pneumocystis jirovecii. With additional data obtained from invivo and invitro studies baicalin and other structurally similar compounds can be considered as useful therapeutic options for Pneumocystis pneumonia patients.
[1]. Smulian AG, Walzer PD, Pneumocystis infections. In: Longo DL, Kasper DL, Jameson JL, Fauci AS, Hauser SL, Loscalzo J, editors Harrison’s Principles of Internal Medicine 2012 18th edUSAMcGraw-Hill:1671-74. [Google Scholar]
[2]. Ewig S, The effect of prophylaxis on the outcome of HIV-associated Pneumocystisjirovecii pneumonia Chest 1996 109:586-87. [Google Scholar]
[3]. Monnet X, Vidal-Petiot E, Osman D, Hamzaoui O, Durrbach A, Goujard C, Critical care management and outcome of severe Pneumocystis pneumonia in patients with and without HIV infection Crit Care 2008 12:R28 [Google Scholar]
[4]. Fei M, Kim E, Sant C, Jarlsberg L, Davis J, Swartzman A, Predicting mortality from HIV-associated Pneumocystis pneumonia at illness presentation: an observational cohort study Thorax 2009 64:1070-76. [Google Scholar]
[5]. Fisk M, Sage E, Edwards S, Cartledge J, Miller R, Outcome from treatment of Pneumocystisjirovecii pneumonia with co-trimoxazole Int. J. STD AIDS 2009 20:652-53. [Google Scholar]
[6]. Mansharamani N, Garland R, Delaney D, Koziel H, Management and outcome patterns for adult Pneumocystis carinii pneumonia, 1985 to 1995: comparison of HIV-associated cases to other immunocompromised states Chest 2000 118:704-11. [Google Scholar]
[7]. Festic E, Gajic O, Limper A, Aksamit T, Acute respiratory failure due to Pneumocystis pneumonia in patients without human immunodeficiency virus infection: outcome and associated features Chest 2005 128:573-79. [Google Scholar]
[8]. Gangjee A, Guo X, Queener SF, Cody V, Galitsky N, Luft JR, Selective Pneumocystis carinii dihydrofolate reductase inhibitors: design, synthesis, and biological evaluation of new 2,4-diamino-5-ubstituted-furo[2,3-d]pyrimidines J Med Chem 1998 41:1263-71. [Google Scholar]
[9]. Anderson AC, Perry KM, Freymann DM, Stroud RM, The crystal structure of thymidylate synthase from Pneumocystis carinii reveals a fungal insert important for drug design J Mol Biol 2000 297:645-57. [Google Scholar]
[10]. Cushion MT, Walzer PD, Ashbaugh A, Rebholz S, Brubaker R, Eynde JJV, In vitro selection and in vivo efficacy of piperazine- and alkanediamide-linked bisbenzamidines against Pneumocystis pneumonia in mice Antimicrob Agents Chemother 2006 50:2337-43. [Google Scholar]
[11]. Cody V, Pace J, Queener SF, Adair OO, Gangjee A, Kinetic and structural analysis for potent antifolate inhibition of Pneumocystis jirovecii, Pneumocystis carinii, and human dihydrofolate reductases and their active-site variants Antimicrob Agents Chemother 2013 57:2669-77. [Google Scholar]
[12]. Porollo A, Meller J, Joshi Y, Jaiswal V, Smulian AG, Cushion MT, Analysis of current antifungal agents and their targets within the Pneumocystis carinii genome Curr Drug Targets 2012 13:1575-85. [Google Scholar]
[13]. Perez-Leal O, Moncada C, Clarkson AB, Merali S, Pneumocystis S-adenosylmethionine transport: a potential drug target Am J Respir Cell Mol Biol 2011 45:1142-46. [Google Scholar]
[14]. Dahlin JL, Kottom T, Han J, Zhou H, Walters MA, Zhang Z, Pneumocystisjirovecii Rtt109, a Novel Drug Target for Pneumocystis Pneumonia in Immunosuppressed Humans Antimicrobial Agents and Chemotherapy 2014 58(7):3650-59. [Google Scholar]
[15]. Rester U, From virtuality to reality - Virtual screening in lead discovery and lead optimization: A medicinal chemistry perspective Curr Opin Drug Discov Devel 2008 11(4):559-68. [Google Scholar]
[16]. Rollinger JM, Stuppner H, Langer T, Virtual screening for the discovery of bioactive natural products Prog Drug Res 2008 65(211):213-49. [Google Scholar]
[17]. Sun H, Pharmacophore-based virtual screening Curr Med Chem 2008 15(10):1018-24. [Google Scholar]
[18]. Kroemer RT, Structure-based drug design: docking and scoring Curr Protein Pept Sci 2007 8(4):312-28. [Google Scholar]
[19]. Colovos C, Yeates TO, Verification of protein structures: patterns of non-bonded atomic interactions Protein Sci 1993 2(9):1511-19. [Google Scholar]
[20]. Ramachandran GN, Ramakrishnan C, Sasisekharan V, Stereochemistry of polypeptide chain configurations J Mol Biol 1963 7:95-99. [Google Scholar]
[21]. Orisis property explorer [Internet]. Cited 2016 February 12. Available from: http://www.organic-chemistry.org/prog/peo/ [Google Scholar]
[22]. Patel N, Koziel H, Pneumocystis jiroveci pneumonia in adult patients with AIDS: treatment strategies and emerging challenges to antimicrobial therapy Treat Respir Med 2004 3(6):381-97. [Google Scholar]
[23]. Chothia C, Lesk AM, The relation between the divergence of sequence and structure in proteins EMBO J 1986 5(4):823-26. [Google Scholar]
[24]. Roy A, Kucukural A, Zhang Y, I-TASSER: a unified platform for automated protein structure and function prediction Nature Protocols 2010 5:725-38. [Google Scholar]
[25]. BLAST Assembled Genomes [Internet]. Cited 2016 February 12. Available from: http://blast.ncbi.nlm.nih.gov/Blast.cgi?CMD=Web&PAGETYPE=BlastDocs&DOC TYPE=FAQ#expect [Google Scholar]
[26]. UCLA-DOE LAB – ERRAT [Internet]. Cited 2016 February 12. Available from: http://services.mbi.ucla.edu/ERRAT/ [Google Scholar]
[27]. Molegro Virtual Docker [Internet]. Cited 2016 February 12. Available from: http://molegro-virtual-docker.software.informer.com/ [Google Scholar]
[28]. The Organic Chemistry of drug design and drug action [Internet]. Cited 2016 February 12. Available from: http://www.organic-c hemistry.org/prog/peo/cLogP.html [Google Scholar]
[29]. Takahashi H, Chen MC, Pham H, Angst E, King JC, Park I, Baicalein a component of Scutellariabaicalensis, induces apoptosis by Mcl-1 down regulation in human pancreatic cancer cells BiochimBiophysActa 1813 8:1465-74. [Google Scholar]
[30]. Zhou L, Zhou BY, Influence of baicalin on TNF-alpha and soluble intercellular adhesion molecule-1 in rats infected with Pneumocystis carinii 2009 27(2):144-147. [Google Scholar]
[31]. Luo J, Kong J, Dong B, Huang H, Wang K, Wu L, Baicalein attenuates the quorum sensing-controlled virulence factors of Pseudomonas aeruginosa and relieves the inflammatory response in P. aeruginosa infected macrophages by down regulating the MAPK and NFκB signal-transduction pathways Drug Design, Development and Therapy 2016 10:183-203. [Google Scholar]