The SARS-CoV-2, a novel coronavirus strain, a new pathogen of the previously known coronavirus family, capable of causing pneumonia of varying severity was first identified in Wuhan, China in December 2019. India has the second-highest number of COVID-19 cases in the world and is recording spikes in numbers every day. Most patients present with mild symptoms and can be better managed with isolation and supportive care [1]. Patients with severe illness worsen rapidly and ultimately die due to respiratory distress, coagulopathy, and metabolic diseases [2]. The early identification and timely treatment of patients with severe disease is crucial in reducing mortality and for effective allocation of medical resources [3]. The NLR is the ratio of neutrophils to lymphocytes, calculated from the routine blood test and is considered to be of great value in indicating a patient’s overall inflammatory status [4].
Elevation of NLR in COVID-19 patients can be an indicator of severe inflammatory progression which could lead to significant complications [5]. Research from several countries has established that NLR aids in risk stratification and is an emerging biomarker [6] of severity and mortality in hospitalised COVID-19 patients. The utility of NLR as a predictor of disease severity in Indian patients with COVID-19 needs to be elucidated. This study attempts to evaluate the effectiveness of NLR in predicting the severity of COVID-19 in a large cohort of Indian patients. The reference value of NLR is expected to vary with age, race and ethnicity and these variations need to be considered while using NLR for diagnostic and predictive purposes in a particular population. Hence, this study also seeks to propose a suitable cut-off of NLR for effective clinical usage to predict severity of COVID-19 in the Indian population.
Materials and Methods
This study was an ambispective observational, single-centre study that included 1100 patients diagnosed with SARS COV-2 infection, treated at Government Tirunelveli Medical College, a tertiary care centre in South India from March 2020 to July 2020. The study was conducted retrospectively from March 2020 to April 2020 and prospectively from April 2020 to July 2020. The study was approved by the Ethics Committee of the Institute (Ref No: 1789/PATH/2020).
Inclusion criteria: The study included 1100 COVID-19 infected patients with throat swabs positive for SARS-CoV-2 RNA by Reverse Transcriptase- Polymerase Chain Reaction (RT-PCR) (Labgenomics RT-PCR kit-Food and Drug Administration (FDA) Approved).
Exclusion criteria: Patients who died on admission, patients who were referred from other centers after initial treatment and patients who were transferred to other hospitals were excluded from the study.
Study Procedure
The nasopharyngeal swabs were collected, transported, stored and processed as per standard protocol. The kits were stored as per manufacture’s instruction. The kit instructions were followed for extraction and amplification of nucleic acids from the samples. The nucleic acids were amplified and SARS-COV-2 was detected using Applied Biosystems® 7500 RT-PCR instrument and the CFX96TM RT-PCR detection system. The tests were performed with appropriate controls. The patients were admitted to the hospital on the day of swab positive results. Diagnosis of COVID-19 infection and clinical classification were done according to “Clinical Management Protocol: COVID-19”, of the Ministry of Health and Family Welfare and Director General of Health Services [7].
The clinical classification is as follows: 1) Mild: Patients with uncomplicated upper respiratory tract infection (may have mild symptoms such as fever, cough, sore throat, nasal congestion, malaise, headache, respiratory rate <24 breath/min, SpO2 >94% in room air); 2) Moderate: Pneumonia with no signs of severe disease (respiratory rate ≥24-30 breath/min, mean oxygen saturation ≤94% (90-94) in room air); 3) Severe: Respiratory distress with mean oxygen saturation <90%, respiratory rate >30 breath/min, respiratory failure with shock, and multiorgan dysfunction. Contacts of patients and patients with travel/exposure history tested throat swab positive by PCR, without any clinical signs like fever sore throat, dry cough, dyspnea was classified as asymptomatic. Asymptomatic and mild cases were given supportive care, antipyretics, and multivitamins. Their saturation and temperature were continuously monitored. Treatment of moderate and severe cases was personalised considering the individual’s clinical status and co-morbidities. It included low molecular weight heparin, remedesivir, methylprednisolone, O2 supplementation and tocilizumab in cases of suspected cytokine storm. The discharge criteria were: 1) Absence of symptoms (afebrile for >3 days without antipyretics and no breathlessness); 2) Maintenance of saturation at room air with no oxygen supplementation for >3 days.
Data Collection
For the patients included in the study, travel history, exposure history, contact history, demographic data, signs, symptoms and laboratory values for the samples collected on the day of admission before initiation of treatment were collected from the hospital records. A 3 mL peripheral venous blood sample was collected as per standard protocol in dipotassium Ethylenediamine Tetraacetic Acid (EDTA) containers and complete blood counts were performed in central diagnostic laboratory using XN2000 (Sysmex Corporation, Kobe JAPAN).
Statistical Analysis
Descriptive analysis was done and data were presented as numbers (percentages) for categorical variables and mean (standard deviation) for continuous variables. Summary statistics of the clinical demographic and laboratory parameters were stratified by NLR tertiles. Statistical significance of the association between demographic, clinical and laboratory data and the tertiles of NLR was analysed using Chi-square test for categorical variables and the difference among the groups for continuous variables was determined using one-way ANOVA. The optimal cut-off value for the continuous variable NLR was calculated by applying ROC analysis, utilising the AUC, sensitivity and specificity. Logistic regression model was generated with NLR cut-off and other influential factors to evaluate their relationship with severity of the infection. The Kaplan-Meier survival function was used to determine the difference in duration of hospital stay of the live patients, based on the NLR cut-off and was considered significant when p<0.05. The relationship of NLR cut-off, and other co-morbidities with mortality was evaluated using Cox regression analysis. All the statistical tests were performed using IBM Statistical Package for Social Sciences (SPSS) software version 25.0.
Results
The study population included 1100 COVID-19 infected cases comprising of 420 (38%) females and 680 (62%) males. Among the total cases, 226 (21%) were mild cases, 27 (3%) were moderate cases, 67 (6%) were severe cases and 780 (70%) were asymptomatic cases. The overall mortality rate was 4.9%. [Table/Fig-1] compares the demographic, clinical and haematological parameters of the study population by tertiles of NLR. Compared with subjects in the lowest tertiles of the NLR, those in the highest tertiles were older and were males. Most of the patients with severe clinical manifestations were in the highest tertile of NLR. Incidence of death increased significantly in the third tertile (1 death in tertile 1 and tertile 2 each vs 52 deaths in tertile 3).
Distribution of demographic characteristics, symptoms, co-morbidity, laboratory findings on the day of admission based on Neutrophil Lymphocyte Ratio (NLR) (n=1100).
Variables | Tertile 1 (0.3-1.23) n=363 | Tertile 2 (1.23- 1.94) n=361 | Tertile 3 (1.94-49.0)n=376 | p-value |
---|
Demographic details | Age* (in years) | 33.24±17.8 | 37.21±15.8 | 44.18±18.3 | <0.001 |
Gender | | | | |
Male | 229 (63.08%) | 221 (61.21%) | 230 (61.17%) | 0.687 |
Female | 134 (36.91%) | 140 (38.78%) | 146 (38.82%) |
Symptoms on admission | Fever | 54 (14.9%) | 46 (12.7%) | 70 (18.6%) | 0.082 |
Dry cough | 31 (8.5%) | 24 (6.6%) | 59 (15.7%) | <0.001 |
Loss of smell and taste | 18 (4.9%) | 16 (4.4%) | 33 (8.8%) | 0.026 |
Gastrointestinal symptoms | 10 (2.8%) | 9 (2.5%) | 16 (4.3%) | 0.337 |
Myalgia | 6 (1.6%) | 4 (1.1%) | 10 (2.6%) | 0.277 |
Sore throat | 13 (3.6%) | 19 (5.2%) | 20 (5.3%) | 0.454 |
Breathing difficulty | 3 (0.8%) | 2 (0.6%) | 51 (13.6%) | - |
Co-morbidities | Respiratory diseases | 3 (0.8%) | 2 (0.6%) | 8 (2.1%) | - |
Diabetes mellitus | 40 (11.0%) | 36 (9.9%) | 71 (18.9%) | <0.001 |
Coronary artery disease | 5 (1.4%) | 2 (0.5%) | 12 (3.1%) | - |
Liver disease | 2 (0.5) | 1 (0.3%) | 5 (1.3%) | - |
Chronic kidney disease | 1 (0.3%) | 1 (0.3%) | 7 (1.9%) | - |
Symptomatic condition | Asymptomatic | 277 (76.3%) | 282 (78.1%) | 221 (58.8%) | <0.001 |
Mild | 81 (22.3%) | 74 (20.4%) | 71 (18.9%) |
Moderate | 2 (0.5%) | 2 (0.6%) | 23 (6.1%) |
Severe | 3 (0.8%) | 3 (0.8%) | 61 (16.2%) |
Duration of hospital stay* (Days) | 9.58±3.5 | 10.58±3.9 | 11±6.9 | <0.001 |
Mortality | Dead | 1 (0.3%) | 1 (0.3%) | 52 (13.8%) | <0.001 |
Alive | 362 (99.7%) | 360 (99.7%) | 324 (86.1%) |
Blood parameters examined at the time of admission | Total WBC count* (109/L) | 7.40±3.5 | 7.77 ±4.21 | 8.82±3.27 | <0.001 |
Neutrophils* (109/L) | 3.15±1.5 | 4.37±2.50 | 6.32±2.85 | <0.001 |
Lymphocytes* (109/L) | 3.59±1.85 | 2.82±1.52 | 1.93±0.85 | <0.001 |
Eosinophils* (109/L) | 0.08±0.05 | 0.07±0.04 | 0.06±0.04 | <0.001 |
RBC count * (1012/L) | 4.85±0.7 | 7.45±34.8 | 5.93±23.7 | 0.860 |
Haemoglobin* (g/dL) | 13.46±2.4 | 13.34±2.2 | 13.02±2.5 | 0.046 |
Packed cell volume* % | 40.03±19 | 38.89±5.1 | 37.73±6.7 | 0.177 |
Platelets* lacs/cumm | 2.87±0.9 | 2.85±0.9 | 2.85±1.0 | 0.384 |
Data are mean±SD; or percentage; The parameters marked with * were continuous variables and one-way ANOVA test was used to find the p-values; whereas other variables are categorical values and Chi-square test was used; The p-value <0.05 was considered statistically significant; WBC count: White blood cell count; RBC count: Red blood cell count
The ROC curve indicated that NLR is a better predictor of severe disease, with 89.3% AUC at the cut-off value of 2.8 with 80.6% sensitivity and 87.1% specificity [Table/Fig-2]. The variables that were significant in univariate analysis were further analysed using logistic regression models to assess the relationship with severity of infection.
ROC curve of Neutrophil Lymphocyte Ratio (NLR) to predict the severity of COVID-19 patients.
At a cut-off 2.8, area under the ROC curve=0.893; 80.6% sensitivity and 87.1% specificity, standard error=0.022; 95% confidence interval=0.849-0.937; p=0.001
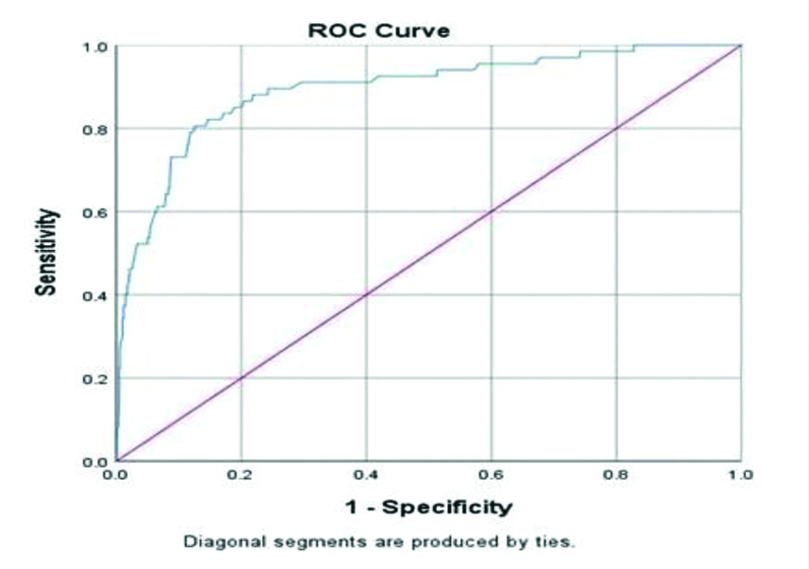
In a logistic regression model generated including NLR >2.8, age >60 years gender, presence of diabetes mellitus, hypertension, patients with NLR >2.8 had the highest odds (OR-19.809, CI-10.159-38.622) of severe COVID-19 infection [Table/Fig-3].
Logistic regression analysing the association of NLR >2.8, age >60 years, gender, presence of dabetes mellitus, presence of hypertension with severity as dependent variable (n=1100).
Parameter | Odds ratio | p-value | 95% Confidence interval lower limit upper limit |
---|
NLR | 19.809 | <0.001 | 10.159 | 38.622 |
Age | 0.161 | <0.001 | 0.083 | 0.312 |
Gender | 1.484 | 0.234 | 0.775 | 2.844 |
Diabetes mellitus | 0.576 | 0.127 | 0.284 | 1.170 |
Hypertension | 0.515 | 0.121 | 0.223 | 1.192 |
NLR: Neutrophil lymphocyte ratio >2.8
Kaplan-meier curves demonstrated significant difference in duration of hospital stay between patients with NLR <2.8 and above NLR >2.8 (p-value >0.001). Patients with NLR <2.8 days were discharged at a mean duration of 10.421 days and NLR >2.8 were discharged at a mean duration of 13.006 days [Table/Fig-4]. The odds of mortality were slightly higher when the NLR was above 2.8 (HR=1.110, 95% CI-1.088-1.113) and this association was statistically significant p<0.001 [Table/Fig-5].
Kaplan-Meier survival curves demonstrating increased duration of hospital stay in patients above NLR 2.8 (Green line) than patients with NLR below 2.8 (Blue line) p-value <0.001.
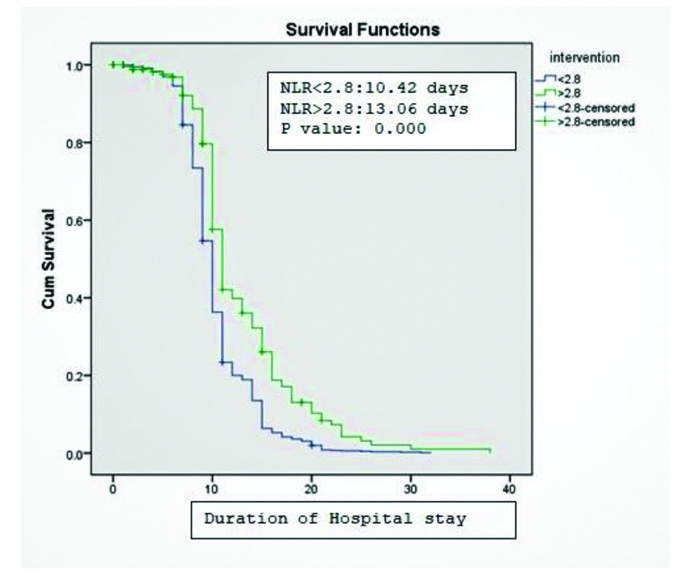
Cox regression analysing the association between NLR >2.8, age >60 years, gender, presence of diabetes mellitus, presence of hypertension with mortality as dependent variable (n=1100).
Parameter | Hazard ratio | p-value | 95% Confidence interval lower limit upper limit |
---|
NLR | 1.110 | <0.001 | 1.088 | 1.133 |
Age | 6.073 | <0.001 | 3.187 | 11.573 |
Gender | 1.031 | 0.913 | 0.595 | 1.788 |
Diabetes mellitus | 2.275 | 0.009 | 1.223 | 4.232 |
Hypertension | 1.759 | 0.103 | 0.892 | 3.468 |
NLR: Neutrophil lymphocyte ratio >2.8
Discussion
Among the 1100 cases, only 6% were severe cases and the predominant population were asymptomatic (70%), mild (21%), and moderate cases (3%). Consistent with published reports [6] elderly men had a higher risk of severe infection. Among the symptomatic patients, fever (53%) and dry cough (35.6%) were the predominant symptoms. The overall mortality in this study was 4.9% which was comparatively higher than, 2.6% mortality reported by Soni L et al., in a study conducted in a tertiary care centre in North India [8]. This study included higher proportion of population above 45 years (36% versus 25.4%) compared to study by Soni L et al., [8]. An upward trend in COVID-19 mortality with age was reported in an epidemiological study conducted in Tamilnadu and Andhra Pradesh by Lakshminarayanan R et al., [9]. Similar to observations by Lakshminarayan R et al., there was a delay in diagnosis of fatal cases in this study and the time to death observed in 50% of fatal cases was less than 48 hours. Thus, the age composition of the cohort, late diagnosis in disease course, and delay in approach to healthcare facility might have contributed to higher mortality rate in this study compared to 2.4% [10] and 2.8% [11] reported in other studies. Similar to recent studies [6], the severity of infection and mortality were higher in patients in the highest tertile of NLR.
Based on ROC curve, a cut-off of NLR=2.8 was found to be 80.6% sensitive and 87.1% specific in predicting severe disease. Patients with NLR >2.8, had higher odds of severe infection and mortality, even after adjusting for age, and co-morbidities like diabetes mellitus and hypertension. Liu J et al., found NLR as the most useful parameter among 26 parameters tested in predicting severe illness [2]. They proposed an NLR value of ≥3.13 and age ≥50 as criteria for stratifying patients into severe phenotype. In a study on hospitalised Chinese patients, Yang A et al., found a higher incidence of severe illness and increased duration of hospital stay in patients with elevated NLR similar to present study findings [12].
The pathogenesis of elevated NLR in COVID-19 patients with severe infection might be enlightened by analysing the causes and consequences of neutrophilia and lymphopenia. The role of neutrophils in viral infections is restricted to early viral defense [13] and is known to contribute to both beneficial antiviral response and detrimental pathology [14]. Gene network analysis revealed direct interaction of the beta coronavirus receptors- Dipeptidyl Peptidase-4 (DPP4), Alanyl Aminopeptidase (ANPEP) with proteins involved in neutrophil response signatures such as neutrophil degranulation [15]. In viral pneumonia, neutrophils play a significant role in the upregulation of chemokine production, reactive oxygen species generation, and recruitment of lymphocytes and monocytes. Coronavirus infected rat models provided histological evidences for the contribution of neutrophil-mediated responses to detrimental lung pathologies like pneumonitis, epithelial necrosis, oedema and vascular leakage in coronavirus infection [14].
The viral particles and the secondary substances derived from the host infected cells are recognised by the pattern recognition receptors on the neutrophils, and they in turn induce Neutrophil Extracellular Traps (NET) formation. The release of NETs amplifies cytokine response, creating a feed-forward loop [16,17]. The filamentous framework of the NETS also facilitates the capture and retention of platelets, coagulation factors, and RBCs, thereby bringing about thrombus formation [18]. An increased serum marker of neutrophil death by NET extrusion (NETosis) is reported recently in hospitalised COVID-19 patients [19]. Thus, we believe neutrophilia as a marker of neutrophil-mediated hyper inflammation.
Insufficient T cell priming due to altered Antigen-Presenting Cells (APC) function and impaired dendritic cell migration was one of the causes of lymphocyte depletion in SARS-COV-2 infection [20,21]. Direct lymphocyte cytotoxicity mediated by Angiotensin-converting Enzyme (ACE) receptor and lymphocyte apoptosis, mediated by TNF and FAS-FAS ligand interaction, may also lead to lymphopenia in COVID-19 infection [22]. Cytokines like IL-6 are also shown to suppress the cytotoxic function of T lymphocytes and natural killer cells and bring about lymphocyte apoptosis [23]. Impairment of T cell expansion and increased sequestration may also contribute to lymphopenia in COVID-19 infection [24,25]. Thus, lymphopenia reflects an insufficient virus-specific immune response.
Thus, it is understood that neutrophilia and lymphopenia are associated with the pathogenesis of severe COVID-19 infection and elevated NLR may indicate the dysregulated immune status of the patient. Therefore, elevated NLR integrates the predictive risk of the two important immune markers into a single risk factor.
The NLR is also a widely evaluated marker for the assessment of the severity of bacterial infections and the prognosis of patients with sepsis [26], pneumonia [27,28], and tumours [29].
The strength of this study is the large sample size hence the observations have sufficient implications in clinical practice. However, this is a single centre study and multicentre studies may aid in validation of our findings, and the proposed NLR cut-off for the Indian population. Further studies on dynamic monitoring of NLR and changes in NLR under treatment, paired with measurement of cytokine levels may strengthen the observations of this study.
Continuous monitoring of oxygen saturation and respiratory rate to diagnose severe infection and instant readiness for respiratory support to prevent death are challenging to an already burdened healthcare system. Therefore, NLR which is cheap and easily calculable could be an initial filter criterion to predict patients at risk of severe infection.
Limitation(s)
The major limitation of this study is that the confounding effects by co-morbidities on haematological parameters could not be eliminated due to incomplete clinical data. This limitation is inherent to hospital-based studies in a pandemic situation. This study did not include dynamic monitoring of NLR.
Conclusion(s)
The heterogeneous presentation of disease severity in COVID-19 infection is due to the unique immune-inflammatory response in every individual. The NLR reflects the dysregulated immune response. The NLR has emerged as a reliable biomarker for disease severity, in various studies across the globe despite the immunological heterogeneity in the study population and our study reconfirms the same in a large cohort of Indian patients. Along with previous international recommendations, this study supports the use of NLR as a readily available and inexpensive biomarker to predict the prognosis of COVID-19 infection.
Data are mean±SD; or percentage; The parameters marked with * were continuous variables and one-way ANOVA test was used to find the p-values; whereas other variables are categorical values and Chi-square test was used; The p-value <0.05 was considered statistically significant; WBC count: White blood cell count; RBC count: Red blood cell countNLR: Neutrophil lymphocyte ratio >2.8NLR: Neutrophil lymphocyte ratio >2.8