Introduction
Cell phone or smart phone abuse has become a daily phenomenon. Many diseases are found to be associated with cell phone overuse. It also leads to mental anxiety and mood disorder.
Aim
To study C-Reactive Protein (CRP), Serum Uric Acid (SUA) and Serum Total Protein (STP) in cell phone addiction and depression together in the medical college campus.
Materials and Methods
The present study was a case-control study in which total 150 subjects with 75 cases of depression and cell phone addiction and 75 healthy controls were involved from medical college of Uttar Pradesh, India. The diagnosis of depression and cell phone addiction was done by (Hamilton, Smart phone Addiction Scale-Short Version) scales. CRP, SUA and STP were quantified using Chem-7 Erba analyser from fasting serum samples. Data was analysed by Statistical Package for the Social Sciences (SPSS) 17.0 software.
Results
Significant correlation between depression and cell phone addiction was found (p=0.023). Significant difference between cases and controls (p<0.001) was found for age, depression score, cell phone addiction score but CRP, SUA and STP were insignificant.
Conclusion
Present research acknowledges many vital observations between cell phone addiction and depression with biochemical trajectories, which is novelty of this study. Youngsters are tending towards addiction and depression, which is an alarm for society and policy makers to rethink about modern gadgets advancements. It is strongly recommended to do further research in this area with more subjects and biochemical markers.
Introduction
In the world of perpetual development of information and technologies, the most dominant type of technological invention is the mobile phone. The consumption and impact of cell phone has grown extensively high in past few years. A cell phone/smart phone works like a portable computer. It gives easy access to information, social connectivity, workplace applications, convenience making it an essential part of our daily lives [1]. It has become a vital device accomplishing abundant functions like internet connection, sending e-mail, purchasing, selling, studying, sensex, putting bids, paying bills, besides these some functions are for entertainment like games, camera, music, movies etc., and definitely affecting personal and social relationships and communications directly or vice versa [2].
Earlier internet was foundation for technological addiction, then came the cell phones which further emerged as a robust source of budding addiction in behaviour, particularly due to smart phone devices [3]. Irrational abuse of smart phones is identified as smart phone addiction by psychologists and is likely to be among the most predominant forms of addictions [4]. According to the latest version of the Diagnostic and Statistical Manual of mental disorders (DSM-5) gambling (behavioural addiction) has been categorised within “substance related and addictive disorders” [5]. It has been verified that smart phone addiction has several similar aspects to DSM-5 substance related disorders including the following four main factors: compulsive behaviour, functional impairment, withdrawal, and tolerance [6].
Sleep insufficiency, anxiety, stress and depression are found to be frequently associated with cell phone usage and internet abuse [3]. Anything that can stimulate a person, first to become habit then converted into an obligation, transforms into an addiction. Consumers have become so dependent on this particular device that they feel inadequate and useless without it, and preoccupancy with the smart phone makes them ignorant about other important stuff [4]. Around the world, smart phones were used by 1.85 billion people in 2014 which is expected to be 2.87 billion in 2020 [7]. According to Newzoo’s Global Report (September 2018) 22.3% of total Indian population are using smart phones. Such huge dependency makes “Cell phone addiction” behaviour a certain [8].
Physical and psychological problems have been observed from cell phone exploitation, which can be muscular pain or stiffness, computer vision syndrome (ocular redness, fatigue, dryness, blurry vision, and irritation), acoustic and tangible illusions- like hearing a ring or feeling a vibration of a cell phone called as ‘Ringxiety’. Pain and weakness in the thumbs and wrists are increasing in number of people [3]. Augner C and Hacker GW revealed significant relation between cell phone abuse, chronic stress and depression in younger females [9]. Hooper V and Zhou Y indicated that stress in students with addiction could be the result of problems derived from cell phone abuse [10]. Excessive and problematic mobile phone use causes dependence as observed by Ahmed I and Qazi TF in their study [11]. Some studies, have reported that problematic mobile phone use causes various mental disorders particularly like depression, anxiety and sleep disorders [12].
Mood disorders of brain affect everyone, irrespective of age, gender, residence and lifestyles, even though some of them are at a higher risk for certain illnesses [13]. Symptoms of depression includes slowing of thoughts, speech and movements, feeling of worthlessness and pessimism in severe cases suicidal thoughts also. It also includes cognitive and emotional areas, starting with mild and progressing into severe levels. Studies have led stress on depression in relation with smart phone abuse [14] but depression and cell phone addiction together is a kind of new virus that is trying to hack every possible mind of the world.
CRP a pentameric protein and frequently studied inflammatory marker. CRP is raised during the acute phase of inflammation. It is an acute phase protein of hepatic origin that increases subsequent after interleukin-6 secretion by macrophages and T-cells. Its role is to bind to lysophosphatidylcholine expressed on the surface of necrotic or apoptic cells or bacteria in direction to activate the complement system thus, promoting phagocytic activity by macrophages, thereby cleansing necrotic, apoptotic cells and bacteria. Howren MB et al., published their meta-analytic study on depression and CRP, launching that depression and CRP were positively associated [15]. Studies have found that subjects with depression and fatigue have higher inflammatory immune activation and fluctuations [16]. In a study by Swartz CM, psychiatric patients showed serum albumin 5.4% lower than non-psychiatric controls [17].
Uric Acid (UA) is the end product of purine metabolism. Endogenous UA is produced by the degradation of nucleic acids, adenine, and guanine by normal or impending dying cells. Exogenous UA is produced by the synthesis of purine by dietary proteins. UA is neuroprotective due to its antioxidant properties which is more than 60% of antioxidant activities in plasma [18]. The chief mechanisms of UA comprises: peroxidase activity maintenance, which in turn prevents the formation of superoxide and peroxynitrite; prevention of cytoskeleton from cellular enzymes which are triggered by peroxynitrite [19]. A study conducted on SUA and serotonin levels in depression analysed that the SUA level of cases was significantly higher than controls [20]. Total protein levels may vary if nutritional status of person is not sufficient, depression can cause symptom of loss of appetite, hence its STP evaluation in depression is imperative. There has been lot of conflicting studies [21,22] regarding depression and biochemical parameters. Until now no researchers have tried to explore the area of cell phone addiction and depression and biochemical parameters simultaneously it may be the first time to be testified. Thus, aim of present study was to find CRP, STP and SUA levels in patients with depression and cell phone addiction and compare with controls.
Materials and Methods
The present study was a observational type of case-control study which conducted in the Department of Biochemistry, of FH Medical College and Hospital Etmadpur, Agra with the collaboration of Santosh Medical College and Hospital Ghaziabad from December 2018 onwards and thesis work is still under progress. Total number of 150 subjects in age range of 18 years and above irrespective of sex which were enrolled according to the protocol criteria of study design, among the staff/students, residents of FH medical college and any patient’s attendants coming in OPD of the medical college. A total of 75 individuals were cases, selected and the other 75 individuals were controls. Sample was taken according to prevalence rate of 4.4% of global population 2015 according to World Health Organisation (WHO), it was calculated by prevalence formula which came out to be 65 required number of cases but to be more effective, 10 extra was taken in each group [23]. All subjects, consent was taken prior to this study. A detailed history of every participant regarding the present or past was taken. This study included staff members, students, residents, patient’s attendants coming in Out Patient Department (OPD) of medical college. All of them were apparently healthy (not suffering from acute infections/fever). Pregnant females, people on any kind of therapy, person with any disorders (diabetes, hypertension, autoimmune diseases, infection, and acute medical or any other surgical condition) were excluded. This study was approved by ethical committee of Santosh Medical College and Hospital, Ghaziabad, Uttar Pradesh (EIC/FN:SU/2018/1456).
Two types of pre-defined and tested scales were used in this study. Evaluation of the smart phone addiction was done by Smart Phone Addiction Scale Short Version (SAS-SV) made by Dr. Kwon and associates. It is a set of 10 questions. The SAS-SV scores of gender and self-evaluation of smart phone addiction (p=0.001) shows a cut-off value of 31 (boys), a cut-off value of 33 (girls) [24]. The depression was evaluated by Hamilton Depression Rating scale (HAM-D), which contains set of 21 questions but only 17 were used as last ones are of extreme depression related. This scale can evaluate various levels of depression depending on scores. Scoring was evaluated as (0-7=Normal, 8-13=Mild Depression, 14-18=Moderate Depression, 19-22=Severe Depression, >23=Very Severe Depression) [25]. During personal interview use of smart phone/cell phone duration was asked to fill on daily basis in hours, this parameter was observed as Time in the result formation. Informed consent, both in English as well as vernacular language, was taken from the participants included in the study. First personal interrogation and both scale study was done and then blood sampling for laboratory investigations was conducted, taking all necessary precautions. The blood sample (approx. 4ml) was centrifuged after clot formation to perform the following biochemical investigations. Biochemical investigations was done after meeting the criteria of this study (Depression and addiction of mobile phone simultaneously). Human CRP-assay kit was used for the quantitative analysis of CRP in human serum. STP and SUA was measured on semi-autoanalyser CHEM 7 by regular kits.
Statistical Analysis
After all data collected the statistical analysis was done using the SPSS 17.0 program (SPSS Inc., Chicago, IL, USA) in Windows 10 Ultimate. The statistical analysis of the data includes descriptive tests and analytical parametric tests, as well as binary logistic regression tests and correlations. The descriptive statistics performed to report the analysis of the data as mean and standard deviations. The independent t-test was used to compare the parametric variables between the genders. Pearson correlation was used to determine the strength of the relationships between the examined variables.
Results
In this study 75 cases and 75 controls subjects analysed the question of research. Cases were those who were addicted to their smart phones/cell phones according to SAS-SV (depicted as SPAS) and were also in depression (on HAMD basis). Group statistics are explained in [Table/Fig-1]. Mean age in cases were 21.68±2.32 years and 28.61±9.97 years among controls. SUA, STP, CRP levels of cases were 5.17±1.72 mg/dL, 7.23±0.82 gm/dL, 2.66±2.79 mg/L, for controls UA, STP, CRP levels 5.48±1.53 mg/dL, 7.18±0.85 gm/dL, 2.56±4.41 mg/L. Average time of cell phone use by all participants in hours was found to be 5.12±2.36 hours, 2.53±1.73 hours among cases and controls, respectively.
Showing demographic and biochemical parameters in cases and controls.
| Series | N | Mean | Std. Deviation | Std. Error mean |
---|
Age (Years) | N | 75 | 28.61 | 9.97 | 1.15 |
C | 75 | 21.68 | 2.32 | 0.26 |
Time (Hours) | N | 75 | 2.53 | 1.73 | 0.2 |
C | 75 | 5.12 | 2.36 | 0.27 |
SPAS (Score) | N | 75 | 17.43 | 6.84 | 0.79 |
C | 75 | 37.75 | 5.06 | 0.59 |
HAMD (Score) | N | 75 | 4.68 | 2.08 | 0.24 |
C | 75 | 12.21 | 3.45 | 0.4 |
UA (mg/dL) | N | 75 | 5.48 | 1.53 | 0.18 |
C | 75 | 5.17 | 1.72 | 0.2 |
STP (g/dL) | N | 75 | 7.18 | 0.85 | 0.1 |
C | 75 | 7.23 | 0.82 | 0.09 |
CRP (mg/L) | N | 75 | 2.56 | 4.41 | 0.51 |
C | 75 | 2.66 | 2.79 | 0.32 |
C: Cases; N: Controls; SPAS: Smart phone addiction score; UA: Uric acid; STP: Serum total protein; CRP: C-reactive protein; HAMD: Depression score
[Table/Fig-2] depicts the Levene’s test for equality of variances and according to t-test, the variable age was found significant. Time spend on smart phone use was statistically significant between cases and controls, but in this study CRP, UA and STP were insignificant between cases and controls when equal variances are assumed.
Comparison between cases and control using Independent t-test.
| Levene’s test for equality of variances | T-test for equality of means |
---|
F | Sig. | T | Df | Sig. (2-tailed) | Mean difference | Std. Error difference |
---|
Age (Years) | Equal variances assumed | 112.52 | 0 | 5.866 | 148 | <0.001** | 6.93 | 1.18 |
Time (Hours) | Equal variances assumed | 2.39 | 0.124 | -7.662 | 148 | <0.001** | -2.59 | 0.34 |
SPAS (Score) | Equal variances assumed | 22.992 | 0 | -20.68 | 148 | <0.001** | -20.32 | 0.98 |
HAMD (Score) | Equal variances assumed | 10.279 | 0.002 | -16.18 | 148 | <0.001** | -7.53 | 0.47 |
CRP (mg/L) | Equal variances assumed | 0.993 | 0.321 | -0.169 | 148 | 0.866 | -0.1 | 0.6 |
UA (mg/dL) | Equal variances assumed | 2.684 | 0.104 | 1.139 | 148 | 0.257 | 0.3 | 0.27 |
STP (g/dL) | Equal variances assumed | 0.396 | 0.53 | -0.389 | 148 | 0.698 | -0.05 | 0.14 |
Independent samples test; C: Cases; N: Controls; SPAS: Smart phone addiction score; UA: Uric acid; STP: Serum total protein; CRP: C-reactive protein; HAMD: Depression score; **highly significant <0.001
Present study had 33 males and 42 females in cases and 43 males and 32 females in controls. [Table/Fig-3] shows statistics of males and females of cases. Mean age (22.55±2.86 years) of males were more than females (21±1.5 years) in this study. Time duration of smart phone was also higher among males than females that are (5.21±2.99 hours, 5.05±1.75 hours, respectively). It shows that means males are using phone for much longer duration. Smart phone addiction score was found higher in females than males (M=37.64±5.05, F=37.83±5.13) as calculated from SAS-SV. HAMD scores were also higher among females (M=11.7±3.34, F=12.62±3.53), which explains females are more in depression in this study. STP was more in males than females (M=7.3±0.85 gm/dL, F=7.18±0.8 gm/dL). SUA levels were higher in males than females (M=6.44±1.46 mg/dL, F=4.18±1.16 mg/dL). CRP levels of male and females are as follows (2.65±2.9 mg/L, 2.67±2.74 mg/L) with females on higher side.
Group statistics- of cases (males vs females).
Series | Gender | N | Mean | Std. Deviation | Std. Error mean |
---|
Cases | Age (Years) | M | 33 | 22.55 | 2.86 | 0.5 |
F | 42 | 21 | 1.5 | 0.23 |
Time (Hours) | M | 33 | 5.21 | 2.99 | 0.52 |
F | 42 | 5.05 | 1.75 | 0.27 |
SPAS (Score) | M | 33 | 37.64 | 5.05 | 0.88 |
F | 42 | 37.83 | 5.13 | 0.79 |
HAMD (Score) | M | 33 | 11.7 | 3.34 | 0.58 |
F | 42 | 12.62 | 3.53 | 0.54 |
UA (mg/dL) | M | 33 | 6.44 | 1.46 | 0.25 |
F | 42 | 4.18 | 1.16 | 0.18 |
STP (g/dL) | M | 33 | 7.3 | 0.85 | 0.15 |
F | 42 | 7.18 | 0.8 | 0.12 |
CRP (mg/L) | M | 33 | 2.65 | 2.9 | 0.5 |
F | 42 | 2.67 | 2.74 | 0.42 |
C: Cases; SPAS: Smart phone addiction score; UA: Uric acid; STP: Serum total protein; CRP: C-reactive protein; HAMD: Depression score; M: Male; F: Female
When levene’s test of equality of variances was used, significant difference was found between age (p=0.004) and SUA (p=0.001). SPAS scores, HAMD score and other biochemical results were not significant in male and female of cases.
[Table/Fig-4] depicts counts and percentages of individuals in various levels of depression. A total of 54 persons (72%) had mild depression, 15 (20%) had moderate depression, 5 (6.7%) were under severe depression category, 1 (1.3%) was in very severe depression.
Frequency distribution in levels of depression by HAMD.
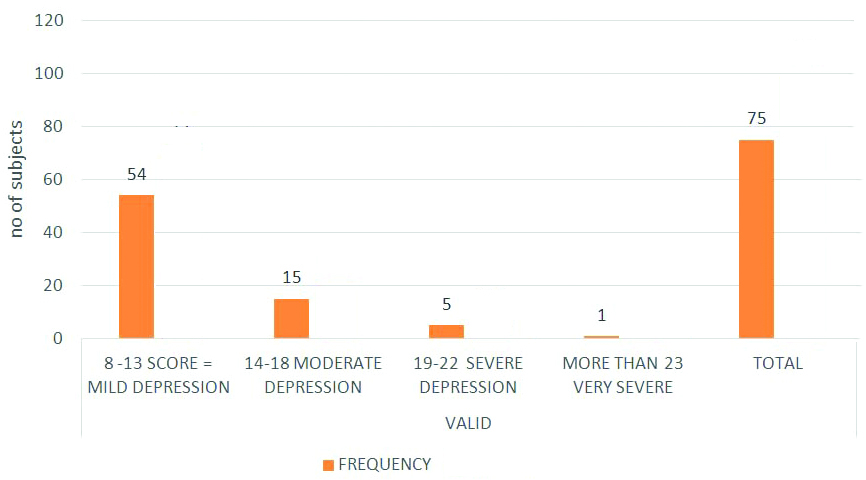
According to HAMD score in this study, depression was categorised. There were 54 cases in mild depression, 15 in moderate depression, 5 cases were of severe depression and only 1 person was in very severe depression in present study. Mean age in cases of mild depression was 21.41±1.654 years, in moderate depression 23.07±3.845 years, in severe and very severe age was 20.8±1.304, 20 years respectively. It was highest among moderate group. Severe and very severe groups had similar means. Age was least in severe and very severe groups of depression, this is an alarming observation. Age was significantly different between cases and controls overall. This is indicating that young generation is tending towards depression. This factor should be investigated thoroughly in other research models as well.
Duration of cell phone use that is observed as Time is highest in very severe depressed person but mild and moderate groups were similar. Time in mild depression was 5.19±2.533 hours, moderate 5±2.035 hours, severe cases had 4.6±1.673 hours and very severe case had 6 hours duration. Mean SPAS score in mild depression was 37.41±5.075, in moderate cases was 36.2±3.052, in severe depressed cases it was 44.8±4.207 and very severe case had 44. Mean SUA levels in mild cases of depression was 5.28±1.75 mg/dL, moderate cases was 4.91±1.48 mg/dL, severe cases had 4.48±2.05 mg/dL and in very severe case was 7 mg/dL. Again SUA levels was also highest in very severe depressed case. Second highest SUA was found in mild group, it could be because this group has highest number of cases.
Mean of STP in mild cases was 7.24±0.75 gm/dL, moderate cases were 7.3±1.02 gm/dL, severe cases were 7.31±0.67 gm/dL and very severe case was 5.3 gm/dL. STP was found to be least in very severe depressed case and it is likely that person is not taking diet on time and according to body needs. CRP levels in mild depression cases was 2.6402±3.031, in moderate depression cases it was 2.794±2.04, severe case 2.516±2.74, in very severe cases it was 2.4. CRP levels were highest in moderate group but lowest in very severe. But since there was only single person in this category, and biochemical parameters were not significant overall also, this could not be made as point of discussion. On applying ANOVA between groups of depression. SPAS scores were found significant between groups of depression where p-value (0.003). This is very important observation in this study. SUA, STP and CRP were not significant between groups.
In [Table/Fig-5] multiple comparison by Tukey HSD (Honestly Significant Difference) was done, significant differences were found for age of mild and moderate groups of depression. SPAS scores were also significant between severe and mild, and severe and moderate depression. CRP, SUA, STP in all categories was insignificant. [Table/Fig-6] presents correlation of all parameters by Pearson correlation. Here age was found to be correlated with TIME (p-value=0.043) and SUA (p-value=0.003). SPAS scores were associated with depression HAMD scores (p-value=0.023). CRP, SUA, STP were not correlated with any parameters.
Multiple comparisons Tukey HSD in mild, moderate and severe forms of depression.
Series | Dependent variable | (I) HAMD | (J) HAMD | Mean difference (I-J) | Std. Error | SIG. |
---|
Cases | AGE (years) | Mild depression | Moderate depression | -1.659* | 0.656 | 0.036* |
Severe depression | 0.607 | 1.051 | 0.832 |
Moderate depression | Mild depression | 1.659* | 0.656 | 0.036* |
Severe depression | 2.267 | 1.161 | 0.132 |
Severe depression | Mild depression | -0.607 | 1.051 | 0.832 |
Moderate depression | -2.267 | 1.161 | 0.132 |
SPAS (score) | Mild depression | Moderate depression | 1.207 | 1.371 | 0.654 |
Severe depression | -7.393* | 2.196 | 0.003** |
Moderate depression | Mild depression | -1.207 | 1.371 | 0.654 |
Severe depression | -8.600* | 2.425 | 0.002** |
Severe depression | Mild depression | 7.393* | 2.196 | 0.003** |
Moderate depression | 8.600* | 2.425 | 0.002** |
CRP (mg/L) | Mild depression | Moderate depression | -0.15381 | 0.830 | 0.981 |
Severe depression | 0.12419 | 1.330 | 0.995 |
Moderate depression | Mild depression | 0.15381 | 0.830 | 0.981 |
Severe depression | 0.278 | 1.470 | 0.98 |
Severe depression | Mild depression | -0.12419 | 1.330 | 0.995 |
Moderate depression | -0.278 | 1.470 | 0.98 |
*The mean difference is significant at the 0.05 level; SPAS: Smart phone addiction score; UA: Uric acid; STP: Serum total protein; CRP: C-reactive protein; HAMD: Depression score
Correlation among different parameters in cases.
Series | Age | Time | SPAS | HAMD | UA | STP | CRP |
---|
Cases | Age (Years) | Pearson correlation | 1 | 0.234* | 0.07 | 0.098 | 0.340** | 0.06 | -0.005 |
Sig. (2-tailed) | - | 0.043 | 0.55 | 0.403 | 0.003 | 0.612 | 0.966 |
N | 75 | 75 | 75 | 75 | 75 | 75 | 75 |
Time (Hours) | Pearson correlation | 0.234* | 1 | 0.196 | 0.028 | 0.111 | 0.021 | -0.173 |
Sig. (2-tailed) | 0.043 | - | 0.092 | 0.809 | 0.342 | 0.856 | 0.138 |
N | 75 | 75 | 75 | 75 | 75 | 75 | 75 |
SPAS (score) | Pearson correlation | 0.07 | 0.196 | 1 | 0.262* | -0.017 | -0.067 | -0.184 |
Sig. (2-tailed) | 0.55 | 0.092 | - | 0.023 | 0.882 | 0.565 | 0.113 |
N | 75 | 75 | 75 | 75 | 75 | 75 | 75 |
HAMD (score) | Pearson correlation | 0.098 | 0.028 | 0.262* | 1 | -0.058 | -0.185 | 0.105 |
Sig. (2-tailed) | 0.403 | 0.809 | 0.023 | - | 0.62 | 0.111 | 0.369 |
N | 75 | 75 | 75 | 75 | 75 | 75 | 75 |
CRP (mg/L) | Pearson correlation | -0.005 | -0.173 | -0.184 | 0.105 | 0.13 | -0.057 | 1 |
Sig. (2-tailed) | 0.966 | 0.138 | 0.113 | 0.369 | 0.266 | 0.625 | - |
N | 75 | 75 | 75 | 75 | 75 | 75 | 75 |
UA (mg/dL) | Pearson correlation | 0.340** | 0.111 | -0.017 | -0.058 | 1 | 0.167 | 0.13 |
Sig. (2-tailed) | 0.003 | 0.342 | 0.882 | 0.62 | - | 0.151 | 0.266 |
N | 75 | 75 | 75 | 75 | 75 | 75 | 75 |
STP (mg/dL) | Pearson correlation | 0.06 | 0.021 | -0.067 | -0.185 | 0.167 | 1 | -0.057 |
Sig. (2-tailed) | 0.612 | 0.856 | 0.565 | 0.111 | 0.151 | - | 0.625 |
N | 75 | 75 | 75 | 75 | 75 | 75 | 75 |
*Correlation is significant at the 0.05 level (2-tailed); **Correlation is significant at the 0.01 level (2-tailed); SPAS: Smart phone addiction score; UA: Uric acid; STP: Serum total protein; CRP: C-reactive protein, HAMD: Depression score
Discussion
A study by Karki S et al., found that the average addiction score among males was 30.23±9.40 and that among females was 28.89±8.63. A higher average addiction score was obtained among males [26]. In present study, males had average addiction score as 37.64±5.05 and females with average score of 37.8±5.13, although not of much difference female scores were higher. Reason behind this attraction may be shopping sites, or social networking/chatting applications as females are already much involved in these activites. A study by Guo N et al., had higher SAS-SV scores for respondents who had symptoms of depression (33.2±10.2 vs. 28.5±10.0; p<0.001) than those with negative screening results [27]. In present study, mobile addiction score for cases vs controls were (37.75±5.06 vs17.43±6.84 p<0.001). Present study proved that depression is in association with mobile addiction. Similarly in study of Dharmadhikari SP et al., out of the 195 students, 90 (46.15%) screened positive for smart phone addiction. These included 45 females and 45 males. An average score of 31.59 (±9.89) by SAS-SV were found [28].
Park SY et al., observed significant changes in the longitudinal studies of relationships among phone use, mobile phone addiction, and depressive symptoms in Korean youth [29]. This was in agreement with present study, where significant correlation was found between depression (HAMD) scores and mobile addiction (SPAS) scores p<0.05 as in [Table/Fig-6]. In [Table/Fig-5], multiple comparisons between severity of depression and mobile addiction were also statistically significant. The possible reason could be intermixed pathways of addiction and depression, anyone can lead to another.
In study of 196 patients with acute ischemic stroke and 100 healthy volunteers; 56 patients (28.6%) were diagnosed for Post Stroke Depression (PSD) by HAMD scale. PSD patients displayed significantly lower levels of UA at baseline as compared to controls [30]. This was similar to present study SUA was lower in cases but not significantly different, possibly due to antioxidant behaviour of SUA.
In study between the First-Episode of Depression (FED) and Recurrent Depressive Disorder (RDD), and healthy controls, no significant differences were observed in reference to acute CRP level and severity of depression. No significant correlation was found between severity of depression and serum concentration of CRP in both the groups [31], this is similar to present study here also CRP levels were insignificant. But in contrast to present study, Köhler-Forsberg O et al., had observed higher and significant association of CRP levels with greater overall Montgomery Aasberg Depression Rating Scale symptom severity (p=0.02), which was significant among women (p=0.02) but not among men (p=0.68) [32]. Also, Song X et al. observed significant differences in total protein (<0.001), albumin, creatinine, UA (<0.001), glucose, creatine kinase and other parameters between the hypertensive depressive cases and only hypertensive [33], which was unlike the present study, where STP was not significant between any groups. Only SUA was found significant between male and females of cases, may be due to similar nutritional status.
Limitation(s)
This study was done with limited sample size and parameters. Authors did not analyse radiations emitted from cell phones which could also have led to draw important conclusion. BMI or other confounders of depression were not investigated in this study.
Conclusion(s)
In this study, a novel approach of finding cell phone addiction and depression together was investigated with biochemical parameters. Astounding results were found, age was one of the significant parameter of this study and decreasing age of addiction and depression in cases is an alarm to society, scientist and technology experts to reframe their strategies. Females are found in more depression with higher level of behaviour addiction compared to males but statistically were insignificant. Depression and mobile addiction was positively correlated. CRP, STP and SUA were not found significant in this study but further researches are required to investigate them. Severity of depression also had severity of mobile phone addiction again who is hunter and who is hunted remains in question. This gives a bidirectional path which has to be investigated with new perspectives.
C: Cases; N: Controls; SPAS: Smart phone addiction score; UA: Uric acid; STP: Serum total protein; CRP: C-reactive protein; HAMD: Depression scoreIndependent samples test; C: Cases; N: Controls; SPAS: Smart phone addiction score; UA: Uric acid; STP: Serum total protein; CRP: C-reactive protein; HAMD: Depression score; **highly significant <0.001C: Cases; SPAS: Smart phone addiction score; UA: Uric acid; STP: Serum total protein; CRP: C-reactive protein; HAMD: Depression score; M: Male; F: Female*The mean difference is significant at the 0.05 level; SPAS: Smart phone addiction score; UA: Uric acid; STP: Serum total protein; CRP: C-reactive protein; HAMD: Depression score*Correlation is significant at the 0.05 level (2-tailed); **Correlation is significant at the 0.01 level (2-tailed); SPAS: Smart phone addiction score; UA: Uric acid; STP: Serum total protein; CRP: C-reactive protein, HAMD: Depression score
[1]. Alosaimi FD, Alyahya H, Alshahwan H, Mahyijari NA, Shaik SA, Smartphone addiction among university students in Riyadh, Saudi Arabia Saudi Med J 2016 37:675-83.10.15537/smj.2016.6.1443027279515 [Google Scholar] [CrossRef] [PubMed]
[2]. Chen YF, Katz J, Extending family to school life: College students use of the mobile phone Human Computer Studies 2009 67(2):179-91.10.1016/j.ijhcs.2008.09.002 [Google Scholar] [CrossRef]
[3]. De-Sola Gutiérrez J, Rodríguez de Fonseca F, Rubio G, Cell-phone addiction: A review Front Psychiatry 2016 7:17510.3389/fpsyt.2016.0017527822187 [Google Scholar] [CrossRef] [PubMed]
[4]. Walsh SP, White KM, Me, my mobile and I: The role of self and prototypical identity influences in the prediction of mobile phone behaviour J Appl Soc Psychol 2007 37:2405-34.10.1111/j.1559-1816.2007.00264.x [Google Scholar] [CrossRef]
[5].  Diagnostic and Statistical Manual of Mental Disorders 2013 5th edWashington DCAmerican Psychiatric Association [Google Scholar]
[6]. Lin YH, Lin YC, Lee YH, Lin PH, Lin SH, Chang LR, Time distortion associated with smartphone addiction: Identifying smartphone addiction via a mobile application (App) J Psychiatr Res 2015 65:139-45.10.1016/j.jpsychires.2015.04.00325935253 [Google Scholar] [CrossRef] [PubMed]
[7]. Cha SS, Seo BK, Smartphone use and smartphone addiction in middle school students in Korea: Prevalence, social networking service, and game use Health Psychology Open 2018 5(1):205510291875504610.1177/205510291875504629435355 [Google Scholar] [CrossRef] [PubMed]
[8]. “Top Countries by Smartphone Penetration & Users-Newzoo”. Retrieved 20 April 2018 [Google Scholar]
[9]. Augner C, Hacker GW, Associations between problematic mobilephone use and psychological parameters in young adults Int J Public Health 2011 57:437-41.10.1007/s00038-011-0234-z21290162 [Google Scholar] [CrossRef] [PubMed]
[10]. Hooper V, Zhou Y, Addictive, dependent, compulsive? A study of mobile phone usage. 20th Bled eConferenceeMergence: Mergin and Emerging Technologies, Processes and Institutions; Jun 4-6; Bled, Slovenia (2007) [Google Scholar]
[11]. Ahmed I, Qazi TF, Mobile phone adoption & consumption patterns of university students in Pakistan Int J Business and Social Sci 2011 2(9):205-13. [Google Scholar]
[12]. Thomée S, Eklöf M, Gustafsson E, Nilsson R, Hagberg M, Prevalence of perceived stress, symptoms of depression and sleep distubances in relation to information and communication technology (ICT) use among young adults- An explorative prospective study Computers in Human Behaviour 2007 23(3):1300-21.10.1016/j.chb.2004.12.007 [Google Scholar] [CrossRef]
[13]. Sharma B, Sharma P, Kumar P, Sharma A, Preclinical concepts of depression Delhi Psy J 2019 22(2):222-31. [Google Scholar]
[14]. American Psychiatric Association Manual for the Definition and Classification of Diseases in Psychiatry (DSM-IV-TR), (Translated by Ertuğrul Köroğlu), Physicians Publishing Union, 4th Edition, Ankara 2001 [Google Scholar]
[15]. Howren MB, Lamkin DM, Suls J, Associations of depression with C-reactive protein, IL-1, and IL-6: A meta-analysis Psychosom Med 2009 71(2):171-86.10.1097/PSY.0b013e3181907c1b19188531 [Google Scholar] [CrossRef] [PubMed]
[16]. Lee CH, Giuliani F, The role of inflammation in depression and fatigue Front Immunol 2019 10:1696Published 2019 Jul 1910.3389/fimmu.2019.0169631379879 [Google Scholar] [CrossRef] [PubMed]
[17]. Swartz CM, Albumin decrement in depression and cholesterol decrement in mania J Affect Disord 1990 19:173-76.10.1016/0165-0327(90)90088-P [Google Scholar] [CrossRef]
[18]. El Ridi R, Tallima H, Physiological functions and pathogenic potential of SUA: A review J Advanced Res 2017 8:487-93.10.1016/j.jare.2017.03.00328748115 [Google Scholar] [CrossRef] [PubMed]
[19]. Pacher P, Beckman JS, Liaudet L, Nitric oxide and peroxynitrite in health and disease Physiol Rev 2007 87:315-424.10.1152/physrev.00029.200617237348 [Google Scholar] [CrossRef] [PubMed]
[20]. Tao R, Li H, High serum uric acid level in adolescent depressive patients J Affect Disord 2015 174:464-66.10.1016/j.jad.2014.12.03125553407 [Google Scholar] [CrossRef] [PubMed]
[21]. Peng YF, Xiang Y, Wei YS, The significance of routine biochemical markers in patients with major depressive disorder Sci Rep 2016 6:3440210.1038/srep3440227683078 [Google Scholar] [CrossRef] [PubMed]
[22]. Chaudhari K, Khanzode S, Khanzode S, Dakhale G, Saoji A, Sarode S, Clinical correlation of alteration of endogenous antioxidant-uric acid level in major depressive disorder Indian J Clin Biochem 2010 25:77-81.10.1007/s12291-010-0016-z23105889 [Google Scholar] [CrossRef] [PubMed]
[23]. Depression and Other Common Mental Disorders: Global Health Estimates. Geneva: World Health Organization; 2017. Licence: CC BY-NC-SA 3.0 IGO [Google Scholar]
[24]. Kwon M, Kim DJ, Cho H, Yang S, The smartphone addiction scale: Development and validation of a short version for adolescents Plosone 2013 8(12):e8355810.1371/journal.pone.008355824391787 [Google Scholar] [CrossRef] [PubMed]
[25]. Hamilton M, A rating scale for depression J Neurol Neurosurg Psychiatry 1960 23(1):56-62.10.1136/jnnp.23.1.5614399272 [Google Scholar] [CrossRef] [PubMed]
[26]. Karki S, Singh JP, Paudel G, Khatiwada S, Timilsina S, How addicted are newly admitted undergraduate medical students to smartphones? A cross-sectional study from Chitwan medical college, Nepal BMC Psychiatry 2020 20(1):9510.1186/s12888-020-02507-132122328 [Google Scholar] [CrossRef] [PubMed]
[27]. Guo N, Luk TT, Ho SY, Lee JJ, Shen C, Oliffe J, Problematic smartphone use and mental health in Chinese adults: A population-based study Int J Environ Res Public Health 2020 17(3):84410.3390/ijerph1703084432013111 [Google Scholar] [CrossRef] [PubMed]
[28]. Dharmadhikari SP, Harshe SD, Bhide PP, Prevalence and correlates of excessive smartphone use among medical students: A cross-sectional study Indian J Psychol Med 2019 41(6):549-55.10.4103/IJPSYM.IJPSYM_75_1931772442 [Google Scholar] [CrossRef] [PubMed]
[29]. Park SY, Yang S, Shin CS, Jang H, Park SY, Long-term symptoms of mobile phone use on mobile phone addiction and depression among Korean adolescents Int J Environ Res Public Health 2019 16(19):358410.3390/ijerph1619358431557844 [Google Scholar] [CrossRef] [PubMed]
[30]. Gu Y, Han B, Wang L, Chang Y, Zhu L, Ren W, Low serum levels of uric acid are associated with development of poststroke depression Medicine (Baltimore) 2015 94(45):e189710.1097/MD.000000000000189726559256 [Google Scholar] [CrossRef] [PubMed]
[31]. Jeenger J, Singroha V, Sharma M, Mathur DM, C-reactive protein, brain-derived neurotrophic factor, interleukin-2, and stressful life events in drug-naive first-episode and recurrent depression: A cross-sectional study Indian J Psychiatry 2018 60(3):334-39.10.4103/psychiatry.IndianJPsychiatry_169_1830405261 [Google Scholar] [CrossRef] [PubMed]
[32]. Köhler-Forsberg O, Buttenschøn HN, Tansey KE, Maier W, Hauser J, Dernovsek MZ, 2017. Association between C-reactive protein (CRP) with depression symptom severity and specific depressive symptoms in major depression Brain Behav Immun 2017 62:34435010.1016/j.bbi.2017.02.02028257825 [Google Scholar] [CrossRef] [PubMed]
[33]. Song X, Zhang Z, Zhang R, Wang M, Lin D, Li T, Predictive markers of depression in hypertension Medicine (Baltimore) 2018 97(32):e1176810.1097/MD.000000000001176830095631 [Google Scholar] [CrossRef] [PubMed]