T2DM is a physical condition characterised by the body’s resistance to INS metabolism or incapability of producing it from the pancreas. It has been reported that, in 2016, 8.5% of the world’s population over 18 years of age suffered from T2DM, compared to 4.7% in 1980 [1,2]. The current treatment strategy solely depends on INS from external sources where the price is always a big issue [3]. Not only the treatment cost but also the economic damage due to the effects on the patient’s health and in productive working hours also need to be reassessed [4]. A number of candidate genes in human accounts for INS resistance; however, Insulin Resistance Gene (INSR) reported to play a major role in INS action and thus categorised as the most sensitive gene for INS metabolism [5]. Mutations in INSR gene have been reported to play a significant role in T2DM in some earlier studies [5,6]. Some of the mutations produce severe and rare INS resistance in humans such as Donohue syndrome and Rabson-Mendenhall syndrome [5,6]. SNPs analysis and understanding of INS and INSR pathways help researchers and clinicians in the development of new drugs and medicines against T2DM [7]. Therefore, other genes in the T2DM pathway could also play a similar role like INS and INSR, as a treatment of INS resistance.
TREH is commonly found in the brush border of the small intestine which hydrolyses a disaccharide, trehalose into glucose subunits. An elevated level of plasma TREH activity has been reported in diabetic patients compared to non-diabetic counterparts [8,9]. Mutations account for a further rise in plasma TREH activity when sometimes, TREH intolerable conditions arise in the small intestine [10]. TREH association with T2DM has been well studied and positive correlation with INS and INSR has also been reported [11,12]. However, identification of pathogenic SNPs in human TREH gene and the possible effects of these mutations on protein functions still remain unstudied. SNPs are the most common genetic variations present in each nucleotide base (A, T, G, and C) of an individual and these variations determine an individual’s susceptibility, drug and immune response to particular diseases. Analysis of non-synonymous SNPs (nsSNPs) alone accounts for 50% of the total genetic differences linked to many diseases [13]. A detailed in-silico analysis of nsSNPs of a specific gene allows us to find out the functional differentiation between true connections and false positive results [14]. Therefore, there is an immense need to sort out the deleterious and damaging nsSNPs from the tolerated one and prioritising them on basis of their effects in molecular and functional level in different datasets as well as how TREH interact with other genes involved in T2DM.
Gene co-expression networking is useful to find out functional association and interaction of genes and for filtering the candidate genes involved in disease association [15]. Correlated genes whereas playing a regulatory role in the metabolic pathway or in a complex protein network [16]. Computational algorithms and online-based tools are frequently used for mapping genes from complex datasets to find out co-relations in the biological pathways [17]. Even though the link between the overexpression of TREH and T2DM has extensively been studied [10], but the pattern of their interactions (TREH and other genes associated with T2DM) and the expression profiling and signalling pathways has been poorly studied.
Laboratory-based analysis of proteins, especially mutation studies required a considerable amount of time. The recent development in bioinformatics tools enables comprehensive analysis of the structural and functional impact of SNPs in the protein stability [18,19]. In addition to mutation studies, computer aided tools frequently opted to find out correlations between genes and proteins in the interacting networks and pathways [20-22]. The aim of the present study was to identify pathogenic missense SNPs in the human TREH gene which have negative impact on protein expression systems and finding functional correlations between TREH, INS, INSR, and other previously reported genes including INS receptor substrate, pro and anti-inflammatory cytokines in T2DM pathways.
Materials and Methods
Site and Length of the Study
A comprehensive in-silico analysis was conducted in the Department of Biotechnology and Genetic Engineering, Bangabandhu Sheikh Mujibur Rahman Science and Technology University, Gopalganj, Bangladesh. The computational analysis lasted for 90 days (September-November, 2019) and data analysis and interpretation required another 60 days (January-February, 2020). An outline of the study design and methods is shown in [Table/Fig-1].
A flow diagram of research methods and computer aided tools used in present study.
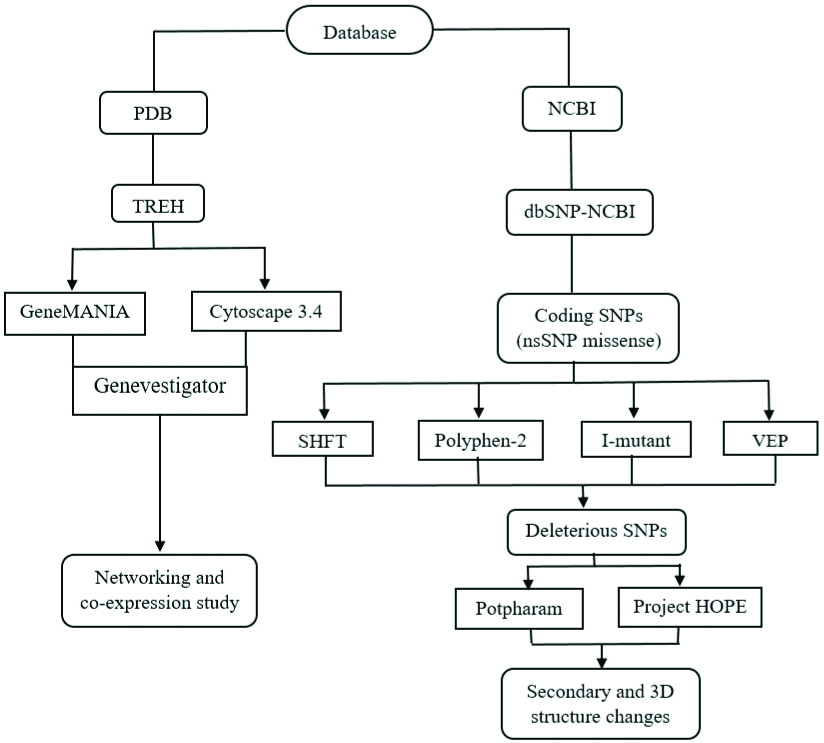
Data Mining
SNPs database (dbSNPs) of National Centre for Biotechnology Information (NCBI) (https://www.ncbi.nlm.nih.gov/snp/) was used for the retrieval of all available SNPs for TREH and related protein information including sequences, chromosome locus, alleles, substituted bases etc. From the dataset, only nonsynonymous (missense) mutations from Homo sapiens were selected for further studies. Missense mutation was selected because, these SNPs reported to change amino acid sequence and have deleterious effect on normal protein function [23]. A total number of 241 nsSNPs in TREH found in the dbSNP database of NCBI were analysed for possible pathogenic SNPs.
Identification of Potential SNPs
A number of computer aided bioinformatics tools are now currently available to decide whether the mutation is neutral or pathogenic, damaging or tolerated. Here, four most commonly used precise tools were used, like Sorting Intolerant from Tolerant (SIFT), Polyphen 2.0, I-mutant, Variant Effect Predictor (VEP) to identify and analyse non-synonymous SNPs in TREH of Homo sapiens.
SIFT (http://sift.jcvi.org/www/SIFT_chr_coords_submit.html) algorithm can precisely predict the amino acid substitution effect on protein stability by filtering deleterious mutations based on tolerance score [24]. Data of nsSNPs from dbSNP database were prepared according to SIFT supported file format of the server. A SIFT score of 0.00-0.05 was regarded as pathogenic or deleterious and above 0.05 was considered as non-pathogenic or tolerated.
Polyphen 2.0 (http://genetics.bwh.harvard.edu/pph2/) is another efficient mutation analysis predictor which combines sequence and structure-based attributes in which the impact of SNPs are calculated by Bayesian Classifier [25]. In this study, queries were submitted as dbSNPs IDs, and Polyphen generate SNPs results either as benign, possibly a damaging, or probably damaging (more confident prediction) based on False Positive Rate (FPR) threshold [13]. A score of 0.5 or less was categorised as tolerated mutation while above 0.5 was considered as damaging effect on protein configuration.
I-mutant (http://folding.biofold.org/i-mutant/i-mutant2.0.html) is a neural web-based Support Vector Machine (SVM), calculating the SNPs and their effect on protein stability. FASTA format of sequence from NCBI dbSNPs was used as input for I-mutant server and result was obtained as increasing or decreasing TREH (PDB code 2JF4) protein stability.
Variant Effect Predictor (https://asia.ensembl.org/info/docs/tools/vep/index.html), the effect of variant (SNPs, insertion, deletion, duplication, substitution) on the structural and functional levels of genes, proteins and regulatory regions [26]. In this study, NCBI nsSNPs IDs were uploaded in VEP predictor and tools SIFT, Polyphen, and Condel were used to predict and calculate the structural and functional changes in the gene. Here, this tool was used for validation of SIFT and Polyphen prediction scores and accuracies.
Predicting the Effects of Deleterious SNPs on Protein Structure
To analyse the impact of deleterious SNPs on protein secondary and tertiary (3D) structure, commonly used web-based server was applied, Portparam (https://web.expasy.org/protparam/) and Project HOPE (http://www.cmbi.ru.nl/hope/method/). Protparam can efficiently calculate different parameters of protein like coil, helices, atomic and amino acid composition, Molecular Weight (MW), theoretical pI, instability index etc. The author inputs the original protein sequence of TREH from PDB (PDB code: 2JF4) and selected SNPs on Protparam in different tabs to analyse the changes in atomic and molecular level due to deleterious mutations found in SIFT, Polyphen, I-mutant, and VEP. Project HOPE is another web based tool to predict tertiary structure changes due to SNPs after gathering all the available data sources. Here, original structure of TREH from protein data bank (PDB code: 2JF4) was uploaded and changed the amino acid with substituted one (ARG by HIS and LEU by ILE) in the desired position. HOPE then determined how much the amino acid changes affect the protein 3D configuration with detailed explanation.
Gene Networking and Regulation Study
GeneMANIA (https://genemania.org/), and Cytoscape 3.4.0 were applied together to find out functional association among TREH, INS, INSR (INS receptor), and PPARG (Peroxisome Proliferator-activated Receptor Gamma) and their role in T2DM. INS, INSR, and PPARG were selected because they have been reported to be associated with obesity and T2DM [27]. GeneMANIA can effectively find out functionally related genes based on the input gene from a large dataset, association on the basis of co-expression, pathways, protein domain similarity and co-localisation [28]. The author inputs TREH, INS, and INSR in search tool as query genes while GeneMANIA generated a network of these three genes on the basis of how they interact with each other during metabolic pathways and gene expression. Cytoscape (https://cytoscape.org/) is a commonly used tool to visualise protein-protein interaction networks in complex metabolic pathway. In this study, Kyoto Encyclopaedia was downloaded for the Genes and Genomics (KEGG) KGML files for T2DM from NCBI Biosystems and then mapped KEGG to Cytoscape 3.4.0. To retain all the information for pathways (i.e., activation, inhibition), enzymes and associated co-factors; some node and edges were reconstructed by keeping major information’s intact. Due to large amount of information on T2DM pathway, only TREH, INS, and INSR interacting network were plotted and drawn on Cytoscape. Correlations and regulation of TREH was analysed using Genevestigator (V3.0) (http://genevestigator.com/gv/) [29]. The Affymatrix Human Genome U133 Plus 2.0 Array dataset was selected for Homo sapiens organism in default parameter. Then TREH gene was selected under gene selection tool and scatterplot log view was selected for experimental regulation of TREH gene. Only highly statistical significant values (>0.005) and fold change (>3.0) results were sorted in this study.
Statistical Analysis
At all stages, p-value of 0.05 was considered as statistically significant. More stringent p-value (p<0.005) and fold changes (≥3.0) were used to find Pearson correlation among TREH, INS and INSR using Genevestigator and cytoscape.
Results
Selection and Analysis of SNPs
In this study, out of 2383 SNPs in human TREH, 241 coding nsSNPs were selected, because most of the deleterious and damaging mutations are reported in this region. nsSNPs are also likely to be disease causing and they alter amino acid position and thus negatively affect the protein structure and configuration. Among the 241 nsSNPs, three mutations were considered to be deleterious according to SIFT and Polyphen score while other seven were found to be tolerated well by the body. In all the three pathogenic SNPs, tolerant index scores were less than 0.03 in SIFT and more than 0.94 in Polyphen. The results supported by I-mutant revealed that protein stability decreased in all of these mutations. SIFT and Polyphen predictions validated using VEP, and also the tool applied for collecting other associated information’s like protein, cDNA and CDS position; codon and amino acids [Table/Fig-2]. Arginine substitutions by cysteine and histidine at position 215, and valine substitution by isoleucine at position 280 were the most damaging mutations predicted by all the tools used in this study. Other two SNPs, rs782373932 and rs782589785 although displayed pathogenic score in SIFT but demonstrated nonpathogenic (tolerated) in Polyphen and VEP predictions.
Status of predicted nsSNPs in TREH using SIFT, Polyphen, I-mutant, and VEP tools.
SNP | Allele | Protein position | Amino acids | SIFT | Poly-phen | I-mutant | Status |
---|
rs200534594 | C/T | 242 | A/T | 0.11 | 0.015 | Stability decreased | Tolerated |
rs367709723 | C/T | 282 | Y/C | 0.15 | 0.011 | Stability decreased | Tolerated |
rs782111382 | C/T | 277 | R/H | 0.10 | 0.033 | Stability decreased | Tolerated |
rs782272013 | C/T | 246 | E/G | 0.09 | 0.053 | Stability decreased | Tolerated |
rs535722007 | A/G | 215 | R/C | 0.01 | 1.000 | Stability decreased | Deleterious/Pathogenic |
rs541953573 | C/T | 215 | R/H | 0.00 | 1.000 | Stability decreased | Deleterious/Pathogenic |
rs781997725 | C/T | 280 | V/I | 0.03 | 0.940 | Stability decreased | Deleterious/Pathogenic |
rs782009103 | C/T | 291 | S/N | 0.08 | 0.100 | Stability decreased | Tolerated |
rs782373932 | A/G | 248 | I/T | 0.03 | 0.049 | Stability decreased | Tolerated (Benign) |
rs782589785 | C/T | 256 | D/G | 0.02 | 0.014 | Stability decreased | Tolerated (Benign) |
Effects of nsSNPs on Secondary and Tertiary Structure of TREH
Protparam analysis of the effects of substituted amino acid on the secondary structure of protein TREH are shown in [Table/Fig-3]. In all three cases, total number of atoms, Molecular Weight (MW), theoretical pI, stability index, and Grand Average of Hydropathicity (GRAVY) values decreased and thus changed the atomic formula of the protein. However, rs535722007 mutation (215, R/C) was the most damaging than the other two SNPs, rs541953573 and rs781997725. In rs535722007, a single amino acid substitution resulted in total number of atoms, including those responsible for hydrogen bonding significantly decreased (14 less H in mutated protein), molecular mass significantly declined (values dropped by 106.1 Da), 24 total atoms reduced, while instability index increased to 50.20 from 48.87, and so as for GRAVY (hydrophilic/hydrophobic value). The changed properties of proteins in the Project HOPE also support Protparam predictions where five features were common for all three pathogenic SNPs: 1) A difference in charge between wild type and mutated amino acid; 2) Charge of the buried wild type residue lost by mutation; 3) Wild type and mutated residue differ in size; 4) Mutant residue is smaller than wild type; and 5) Mutation will cause an empty space in the core of the protein. Whereas, rs535722007 SNP in Project HOPE had two additional properties: 1) The hydrophobicity of the wild type and mutated residue extremely differ, and more importantly; 2) The mutation will cause loss of hydrogen bonds in the core of the protein that results in incorrect protein folding. Therefore, it can be concluded that rs535722007 SNP is the most damaging mutation which destabilises protein configuration. The wild and mutated protein structures for all three SNPs generated by Project HOPE are shown in [Table/Fig-4].
Effect of deleterious SNPs on secondary structure of TREH.
SNPs | Formula | Total atom | Molecular WT | Theoretical pl | Instability index | GRAVY |
---|
2JF4 (Normal) | C2700H4120N734O818S16 | 8388 | 60463.80 | 5.36 | 48.87 | -0.653 |
rs535722007 | C2694H4106N728O816S17 | 8364 | 60357.70 | 5.23 | 50.20 | -0.627 |
rs541953573 | C2697H4108N730O818S17 | 8370 | 60391.71 | 5.28 | 49.35 | -0.637 |
rs781997725 | C2697H4117N733O818S16 | 8385 | 60410.75 | 5.29 | 49.15 | -0.650 |
Close view of deleterious SNPs in TREH. The protein has been demonstrated in grey colour, the side chains of wild-type and the mutant residue are shown and displayed in green and red colours, respectively. (a) rs535722007 (R/C); (b) rs541953573 (R/H); (c) rs781997725 (V/I). The image generated in Project HOPE.
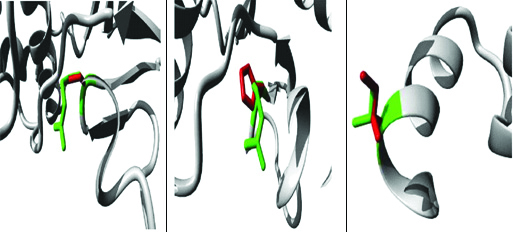
Gene Networking and Regulation Studies
GeneMANIA visualisation of the interacting network among TREH, INS, INSR and PPARG are shown in [Table/Fig-5]. TREH co-expressed with INS and PPARG, and geneNOX4, where NOX4 has strong pathway connection with INS and INSR. Correlation study revealed gene from glucose metabolism pathway like glycosidase, aldolase, phospholipase etc. are the closest neighbour of TREH [Table/Fig-6]. Analysis of KEGG T2DM pathway on Cytoscape also showed a connection with INS resistance to trehalose, substrate where trehalose works. TREH works either through Calcium2+ dependent PKC pathway or DNA mediated PDX-1/MAFA pathway to produce T2DM [Table/Fig-7]. Both pathways impaired normal INS secretion and produced transient hyperglycaemia and then T2DM. INS and INSR may also associated with T2DM through Ca2+ mediated PKC and apoptosis, by the first pathway of TREH, involved in INS resistance. In this study, gene expression revealed down-regulated expression of TREH for most of the human diseases that are linked to INS and INSR, especially in kidney diseases and obesity [Table/Fig-8].
Predicted, co-expression and pathway interaction between TREH, INS, INSR, and PPARG. Yellow line showed predicted links, purple represents co-expression, and sky blue denotes pathway connection between genes.
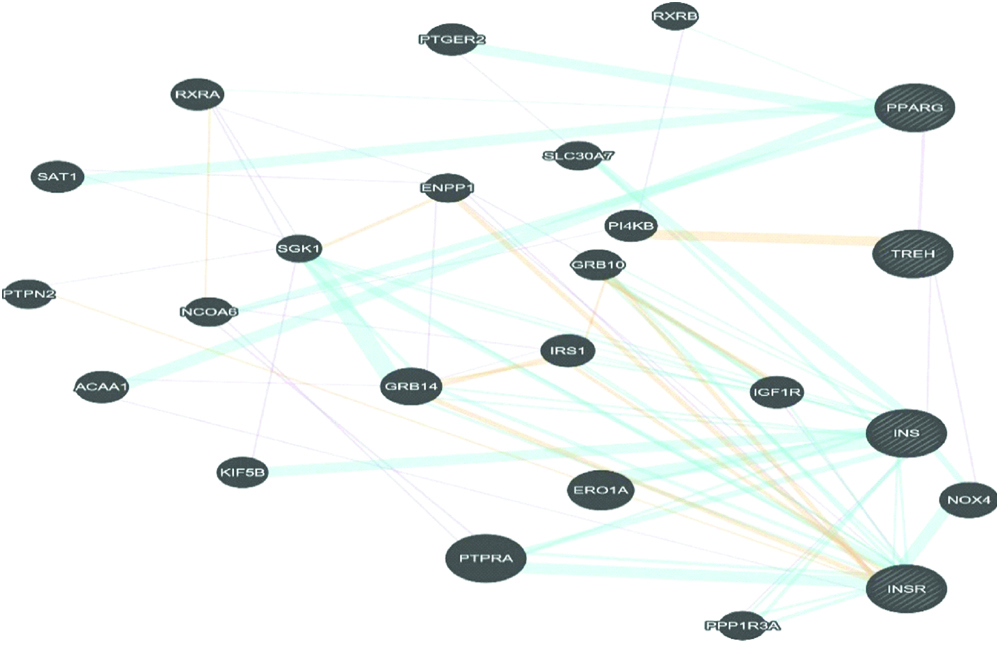
Most correlated gene of human TREH. Black circle at middle is the TREH gene and the correlation values presents as Pearson’s coefficient. The more adjacent to TREH shell means the higher relationship between genes.
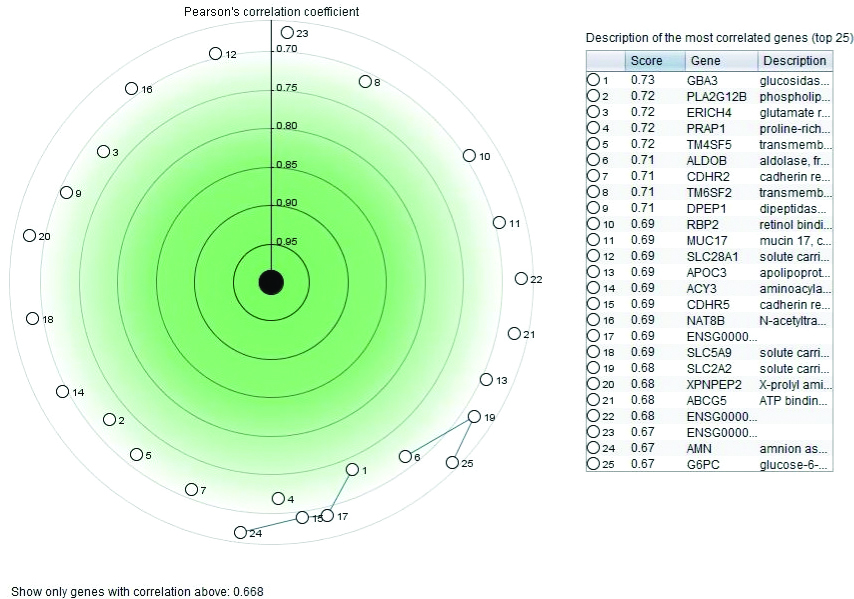
Cytoscape mapping of KEGG pathway for T2DM showing relationship between TREH, INS, and INSR.
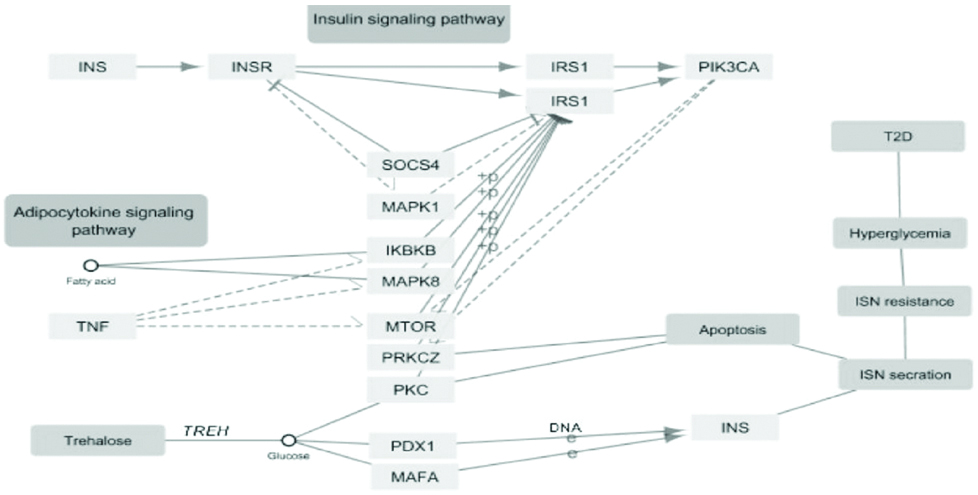
Regulation of human TREH gene expression in eight perturbation dataset. Experimental researches showed that except one, TREH down-regulated in other seven studies, all of which linked with kidney diseases, pancreatic cancer and obesity. The image is generated in conditional search tool from Genevestigator.
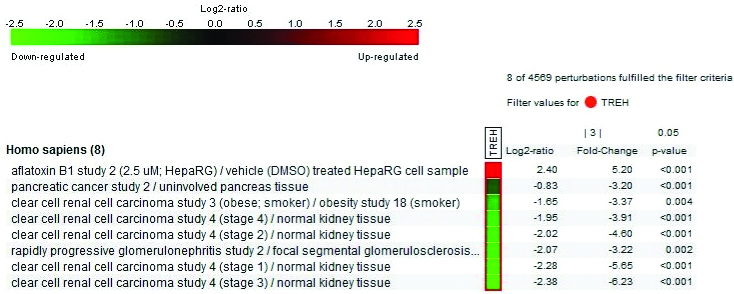
Discussion
TREH is a disaccharide found in human tissue and plasma which works on trehalose in carbohydrate metabolic pathways. Earlier experiment revealed statistically significant association between TREH and diabetes where three SNPs (rs2276064, rs117619140 and rs558907) with high TREH activity were found to be associated with T2DM [10]. Research also found deleterious SNPs results in destabilisation of INSR associated with impaired INS secretion from pancreas [4]. Numerous laboratory studies have been conducted to find out the relationship of plasma TREH level and T2DM as well as potential genes those play functional role in impaired glucose metabolism pathways [30,31]. However, no comprehensive in-silico analysis yet has been done, addressing the impact of deleterious SNPs on functional activity of TREH and how this gene involve in INS resistance. Hence the authors used bioinformatic tools and sequence databases to predict and classify the tolerant and damaging nsSNPs in human TREH and its functional association with INS and INSR.
In the present study, a combination of both sequence and structure based methods for SNPs study were applied. The sequence based studies are only applicable for proteins with unknown 3D structure while studies on tertiary configurations give an overview of changes inside a molecule [32]. In this study, SIFT, Polyphen-2, I-mutant, and VEP sequence mediated tools produced negligible variation of score to classify the SNPs from one group to another (i.e., tolerated to damaging): a prediction always anticipated in case of in-silico study of SNPs [33]. Secondary structure analysis of deleterious TREH SNPs; rs535722007, rs535722007, and rs541953573 revealed R215C as the most destructive mutation that resulted in abrupt atomic and molecular changes in experimental TREH protein. Substitution of arginine by cysteine cause rapid fall in atom and hydrogen molecule, and sharp increase in protein instability index. Hydrogen bonds involve in correct protein folding, rigidity of the structural assembly, molecular recognition and stabilise intermolecular interaction [34]. The 3D structure investigation in rs535722007 SNP also reflects all the previous calculations of damaging effects. Predictions found R215C SNP in TREH cause loss of hydrogen bonds triggering the instability of protein. Protein stability although can be improved in recommended storage condition and laboratory settings [35]. However, some critical amino acids are crucial for stability, and thus, changes in those residues may result in unstable proteins more vulnerable to degradation [36]. Arginine (R) is one of the major amino acid that stabilise protein from aggregation, especially during protein refolding [37]. Several laboratory research studies have been conducted for increasing the thermal stability of protein by replacing other amino acids by arginine [38,39]. Hence, substitution of arginine surely affects diverse protein properties including stability and function.
Networking or clustering of genes is very crucial in understanding the genetic association and molecular mechanism of a disease. The study of gene-gene networking in many cases is likely to be useful to uncover the significant association between other functionally related genes and their subnetworks in the pathway of a particular disease [20,40]. A strong relationship exists between a pair of genes with a similar expression pattern, because they are controlled by same transcriptional factors, regulators and more importantly they play same functions in a pathway or a protein complex [41]. In this study, it was found that TREH, INS, and PPARG are co-expressed and thus perform same functional role in T2DM pathway. Like TREH, the overexpression of PPARG in T2DM progression has already been revealed [27]. Present study also found a strong link between many of the co-related genes of TREH associated with T2DM. Overexpression of apoB-100 is associated with T2DM, where apoB-100 was found to trigger coronary artery calcification during T2DM, and thus reported that measuring the level of apoB-100 is useful in the diagnosis of cardiovascular risk in T2DM [42,43]. A genome wide association studies identified potential SNPs variants for diabetes and cataract located in Glucocerebrosidase-3 (GBA3) and PPAR [44]. The relationship is more supported by KEGG pathway analysis for T2DM, where TREH and INS, both have similar effect on INS secretion but in a different pathway [Table/Fig-7], where INS activate INSR. In addition to that, present study on experimental gene regulation (perturbation) of TREH in Homo sapiens found down-regulation of TREH in all cases of obesity and kidney diseases [Table/Fig-8]; alike research outcomes for experimental therapy against T2DM with INS receptors [45,46]. Therefore, a functional association exist between TREH, INS, and INSR during T2DM progression in humans.
Overall findings suggest that TREH has crucial role in INS resistance pathway and mutations in TREH gene can produce severe INS resistance in humans. However, further laboratory trial is required to analyse the effects of deleterious SNPs in mouse model.
Limitation(s)
Present study deals with a limited number of samples from NCBI dbSNPs database. The findings could be more interesting with combinations of other databases like PBD, UniPortKB and genome-wide screening of SNPs.
Conclusion(s)
In a nutshell, the study effectively used computational biology tools in finding and filtering the most damaging SNPs in TREH. In addition, networking bioinformatics discovered that, TREH and INS both have many correlated genes; co-expressed and are down-regulated in experimental therapy for T2DM, TREH, INS, and INSR, differentially expressed but play similar functions in T2DM pathway. Further genome wide association studies and laboratory trials with mouse model are required for in depth analysis of deleterious SNPs in TREH gene.