Acute cholecystitis is one of the most frequent problems that require emergent surgery [1]. This disease condition is diagnosed through medical history, physical examinations, laboratory findings and imaging results. These are also important for surgical decision making. Acute cholecystitis encounters severe complications such as haemorrhage, gangrene, emphysema, xanthogranuloma, and perforation [1]. Among these complications, gangrenous cholecystitis accounts for 30% of patients admitted to hospital with acute cholecystitis [1]. Teefey SA et al., found that 48% of patients are diagnosed with gangrenous cholecystitis on histopathologial diagnosis and there was no evidence which suggests disease progression pre-operatively [2].
Tokyo Guidelines of 2007 and updated version in 2013 (TG13) recommend a diagnostic criteria for severity grading of acute cholecystitis and cholangitis. TG13 uses clinical features, blood chemistry findings and abdominal ultrasound sonography to classify acute cholecystitis into three grades [3-5]. The patients of gangrenous cholecystitis have similar clinical presentations with acute cholecystitis. Therefore, it is difficult to discriminate between acute and gangrenous cholecystitis. Moreover, gangrenous cholecystitis has been associated with higher morbidity and mortality rate (15-50%) as compared to uncomplicated acute cholecystitis; mainly in elderly, diabetic and immuno-compromised patients [6,7]. To minimise such risks, it would be highly advantageous to predict the severity pre-operatively. Hence, the present study aimed to develop models for prediction of gangrenous cholecystitis according to pre-operative patient-dependent and clinical risk factors.
Materials and Methods
This was a retrospective observational study. We reviewed data of the patients who underwent laparoscopic cholecystectomy due to acute/gangrenous cholecystitis at the tertiary care center, between February 2015 and November 2017. This study was approved by the institutional review board (Protocol no: SIDS-17-01) of the study institution. During this study period, a total of 562 laparoscopic cholecystectomies were performed.
We included patients who were diagnosed with acute or gangrenous cholecystitis on surgical and pathologic examinations. However, we excluded patients whose surgical and pathologic results indicated chronic cholecystitis (n=114) or the presence of malignancy (n=11). Thus, the remaining 437 patients were included in the study.
Laparoscopic cholecystectomy was performed with the standard four-port method. The pre-operative diagnosis of acute cholecystitis was based on clinical and laboratory signs (radiological findings); (presence of right upper quadrant or epigastric pain and tenderness with or without fever and leucocytosis). Upper abdominal ultrasonography or CT scan was used to confirm acute cholecystitis on the basis of findings which showed thickening, and/or oedema of the Gallbladder (GB) wall by a gallstone and the presence of pericholecystic fluid. We stratified the study population into two groups based on operational details and pathologic characteristics: Acute cholecystitis and Gangrenous cholecystitis.
For each patient, the medical record was analysed for the following data: demographics (age, gender), habits (smoking, tobacco chewing and alcohol abuse), pre-existing co-morbidities (hypertension, diabetes, ischemic heart disease), pre-operative clinical manifestation (right upper quadrant abdominal pain, nausea, vomiting, obstructive jaundice, pancreatitis, peritonitis), pre-operative laboratory examination {total White blood cell (WBC) count, aspartate transaminase, alanine transaminase, total bilirubin, and creatinine}, and pre-operative radiological findings (GB wall thickness and pericholecystic fluid collection).
Statistical Analysis
Univariate analysis was performed for the surgical as well as histological diagnosis, as the categorical variables were compared using the Chi-square test. The p-value, Odds Ratio (OR) and 95% Confidence Interval (CI) were determined for each variable. The variables with p-value 0.05 or less were selected for multivariate analysis. Multiple logistic regression analysis was performed to identify pre-operative variables independently associated with gangrenous cholecystitis and to determine those variables independently predictive of gangrenous cholecystitis. Based on that the significant (p-value ≤0.05) variables in the multivariate analysis, predictive models for gangrenous cholecystitis was developed. The statistical analysis was performed using SPSS version 15.
Results
A total of 437 patients (mean age 54.7±15.5 years, 260 males) were included in the study. Of these 286 patients (65.4%) exhibited acute cholecystitis and 151 patients (34.6%) exhibited gangrenous cholecystitis on microscopic examination. All patients underwent ultrasonography and 117 patients (26.8%) underwent CT-scan also. Out of these 117 patients, 50 patients had acute cholecystitis and 67 patients gangrenous cholecystitis. Total 71.6% gangrenous cholecystitis patients were misdiagnosed with acute cholecystitis on CT-scan finding.
Demographic and clinical characteristics of each group are elucidated in [Table/Fig-1]. Univariate analysis identified 13 variables with a p-value less than 0.05. These identified variables were: age, male, diabetes mellitus, hypertension, ischemic heart disease, alcohol abuse, peritonitis, GB wall thickness, pericholecystic fluid, leukocytosis, hyperbilirubinemia, aspartate transaminase, creatinine [Table/Fig-2]. The results of multivariate logistic regression analysis are elucidated in [Table/Fig-3]. The multivariate selection criteria identified 13 variables on univariate analysis. On multivariate analysis, four variables were identified which were independently associated with gangrenous cholecystitis: the presence of diabetes mellitus, laboratory examinations of leukocytosis, and radiologic findings of pericholecystic fluid collection and GB wall thickening.
Summary of the baseline characteristics of study population.
Variables | Cut-off | Acute cholecystitis (n=286) | Gangrenous cholecystitis (n=151) |
---|
Age (years) | <60 | 191 (66.7%) | 84 (55.6%) |
≥60 | 95 (33.2%) | 67 (44.4%) |
Gender | Male | 154 (53.85%) | 106 (70.20%) |
Female | 132 (46.15%) | 45 (29.80%) |
Co-morbidity | DM | 63 (22.1%) | 50 (33.1%) |
Hypertension | 91 (31.9%) | 69 (45.7%) |
IHD | 23 (8.1%) | 23 (15.2%) |
Habits | Smoking | 8 (2.8%) | 3 (2%) |
Tobacco chewing | 11 (3.8%) | 4 (2.6%) |
Alcohol abuse | 02 (0.7%) | 06 (4%) |
Clinical signs | Obstructive jaundice | 95 (33.3%) | 64 (42.4%) |
Peritonitis | 3 (1.1%) | 7 (4.6%) |
Pancreatitis | 50 (17.5%) | 6 (4%) |
DM: Diabetes mellitus; IHD: Ischemic heart disease
Univariate analysis of pre-operative variables.
Variables | Cut-off | Odds ratio (95% CI) | p-value |
---|
Age | <60 years | 1.8 (1.08-2.43) | <0.001 |
Gender | Male | 2.0 (1.34-3.09) | <0.001 |
Alcohol abuse | Present | 5.9 (1.17-29.4) | 0.026 |
DM | Present | 0.573 (0.36-0.89) | 0.014 |
Hypertension | Present | 1.8(1.20-2.69) | 0.005 |
IHD | Present | 2.1(1.11-3.79) | 0.024 |
Peritonitis | Present | 0.219 (0.06-0.86) | 0.027 |
WBC | >10000/mm3 | 0.316 (0.21-0.48) | <0.001 |
Creatinine | >0.8 mg/dL | 0.545 (0.36-0.82) | <0.001 |
Total bilirubin | >1.2 mg/dL | 0.140 (0.08-0.24) | <0.001 |
AST | >30 U/L | 6.6 (4.26-10.35) | <0.001 |
GB wall thickness | >3 mm | 0.087 (0.05-0.11) | <0.001 |
Pericholecystic fluid | Present | 10.97 (6.64-18.1) | <0.001 |
DM: Diabetes mellitus; IHD: Ischemic heart disease; WBC: White blood cells; AST: Aspartate transaminase; GB: Gallbladder
p≤0.05
CI: Confidence interval
Multivariate logistic regression analysis of factors determining gangrenous cholecystitis.
Variables | Ba | p-value | Exp (B)b (Odds ratio) | 95% CI for Exp (B) |
---|
Lower | Upper |
---|
Diabetes mellitus | 0.821 | 0.042 | 2.27 | 1.030 | 5.010 |
White blood cells | -1.190 | 0.001 | 0.304 | 0.158 | 0.585 |
GB wall thickness | -2.694 | 0.001 | 0.068 | 0.032 | 0.141 |
Pericholecystic fluid | 1.666 | 0.001 | 5.289 | 2.743 | 10.199 |
Constant | 0.683 | 0.447 | 1.980 | - | - |
GB: Gallbladder
aLogistic regression equation predicts the log odds, the coefficients (column B) represent the difference between the log odds, a log odds ratio
bThe antilog of the coefficients
p≤0.05
CI: Confidence interval
For quicker and more convenient use in clinical settings, we developed two predictive models based on the multivariate analysis [Table/Fig-3]; the logarithmic values of the OR were calculated. Pre-operative characteristics of diabetes mellitus, pericholecystic fluid collection, thicken GB wall (>3 mm) and leukocytosis (>10000/mm3) was coded as 1 (if present) or 0 (if absent). A standard predicting model was developed to predict gangrenous cholecystitis in symptomatic patients. The probability of gangrenous cholecystitis in symptomatic patients can be calculated using the following formula:
The probability of gangrenous cholecystitis=1/1+e-z (Equation 1)From the results of the multivariate analysis Equation 1 is derived [Table/Fig-3] and ‘z’ is calculated with the help of following formula:
z=0.683-2.694* GB wall thickness (1)+1.666* Pericholecystic fluid (1)+0.821 DM (1)-1.190* WBC (1) (Equation 2)A Receiver operating characteristic (ROC) curve was plotted to determine the cut-off for risk assessment values which could be differentiated between acute and gangrenous cholecystitis [Table/Fig-4]. The ROC area under the curve was 0.908 which indicate in almost 90.8% of all possible pairs of the patient in one has acute cholecystitis and the other have gangrenous cholecystitis. This model assigns the higher probability of patients with gangrenous cholecystitis. The optimal sensitivity and specificity were 82% and 82.2%, respectively [Table/Fig-4].
Receiver operating characteristic (ROC) curves for prediction of gangrenous cholecystitis in the symptomatic patient. The area under the curve: 0.908.
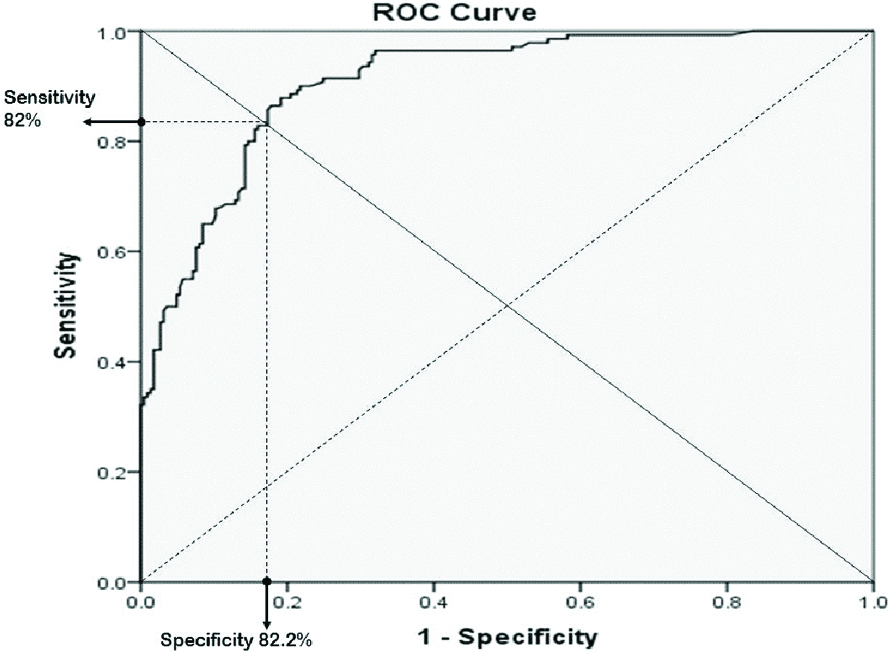
Furthermore, we developed an additive quick-and-easy model based on radiologic findings of pericholecystic fluid collection and GB wall thickness. These both variables are easy to obtain from radiological reports and the same coding of the first model was used. With the help of following formula, we can predict the probability of gangrenous cholecystitis in asymptomatic patients:
Probability of gangrenous cholecystitis in asymptomatic patients=1/1+e-z (Equation 3)Equation 3 was developed from multivariate analysis results [Table/Fig-5] and ‘z’ can be calculated using the following formula:
z= -0.249-2.444* GB wall thickness (1)+1.766* Pericholecystic fluid (1) (Equation 4)ROC curve was plotted and area under the curve was 0.861 and optimal sensitivity and specificity were 78.2% and 78% respectively [Table/Fig-6].
Predictive model of gangrenous cholecystitis for asymptomatic patients.
Variables | Ba | p-value | Exp (B)b(Odds ratio) | 95% CI for Exp (B) |
---|
Lower | Upper |
---|
Pericholecystic fluid | 1.766 | 0.001 | 5.850 | 3.322 | 10.302 |
GB wall thickness | -2.444 | 0.001 | 0.87 | 0.46 | 0.162 |
Constant | -0.249 | 0.228 | 0.780 | - | - |
GB: gallbladder
aLogistic regression equation predicts the log odds, the coefficients (column B) represent the difference between the log odds, a log odds ratio
bThe antilog of the coefficients
p≤0.05
CI: Confidence interval
Receiver operating characteristic (ROC) curves for prediction of gangrenous cholecystitis in asymptomatic patients. The area under the curve: 0.861.
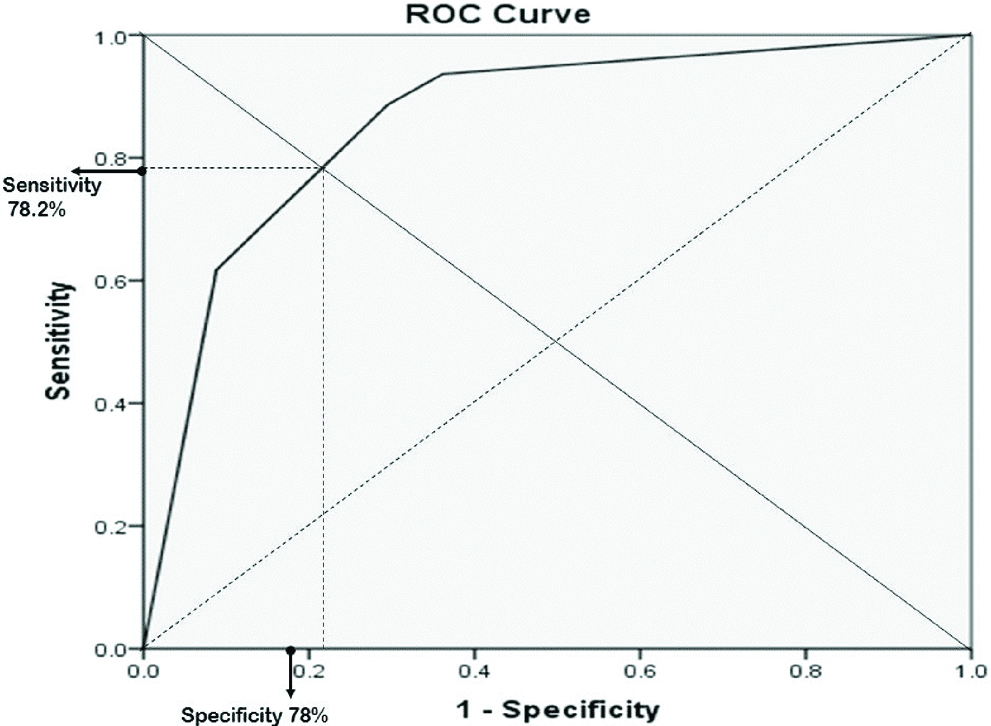
Discussion
Cholecystitis is accurately diagnosed through abdominal CT-scan; however, it still has low accuracy in the differentiation of acute and gangrenous cholecystitis. Bennett GL et al., reported 29.3% of sensitivity and 64.1% of accuracy for diagnosis of gangrenous cholecystitis [8,9]. Hence, this study was aimed to improve the preoperaive predictability of gangrenous cholecystitis. These predictive models were developed to estimate the severity of inflammation by computing patient-dependent risk factors and clinical parameters. The identified patient-dependent risk factor was co-morbidity i.e. diabetes mellitus and clinical parameters were GB wall thickness, pericholecystic fluid collection, and WBC. These parameters were used to develop predictive models of gangrenous cholecystitis. The indexed predictive models showed higher predictability for gangrenous cholecystitis (79% and 82%) than CT-scan (64.1%).
Several attempts have been made to predict gangrenous cholecystitis pre-operatively. However, most of the studies had a small population ranging between 50 to 100 which was the major limitation of all those studies [1,7,10-12]. Yacoub WN et al., attempted to identify pre-operative clinical predictors for gangrenous cholecystitis in 245 patients presented with acute cholecystitis. In their study, they identified age >45 years, male gender, heart-rate >90 beats/min, WBC >13000/mm3 and GB wall thickness >4.5 mm as an independent risk-factors for gangrenous cholecystitis [13]. Similarly, TG13 guidelines uses clinical data findings from blood chemistry and abdominal ultrasound to classify cholecystitis. TG classified cholecystitis into three categories: Grade I, Grade II and Grade III cholecystitis. As per TG13, grade II cholecystitis defines acute cholecystitis as the degree of inflammation that is most likely to be associated with increased operative difficulty during cholecystectomy. The inclusion criteria for grade II cholecystitis by TG13 was marked local inflammation. In marked local inflammation, TG13 includes the presence of gangrenous cholecystitis, pericholecystic abscess, hepatic abscess, biliary peritonitis or emphysematous cholecystitis. Aforementioned parameters could be estimated through the imaging studies pre-operatively [3-5]. TG13 guidelines have been well-established for a while now. However, their validation is not completed. Besides, patient’s co-morbidity, Body Mass Index (BMI) and gender were not considered in the guidelines. These factors directly contribute to the high degree of divergence in the clinical presentation of patients with acute cholecystitis and therefore, these factors should be considered to predict the pre-operative severity of cholecystitis. Moreover, Kim KH et al., and Ambe PC et al., given risk assessment scoring system for differentiation of severe cholecystitis from simple cholecystitis [14,15]. The sensitivity of Kim KH et al., proposed scoring system is 74.5% [14].
For an example: calculating the probability of gangrenous cholecystitis in the symptomatic patient using the standard predictive model. A 67-year-old male presented with right upper quadrant pain. His laboratory examination was normal except leukocytes 14000/mm3 and bilirubin 1.43 mg/dL. Abdominal CT scan revealed acute cholecystitis without pericholecystic fluid collection and 6 mm thick GB wall. Using standard predicting model first we can calculate ‘z’. As a patient has leukocytosis and thicken GB wall, those will be coded with ‘1’ and rest of normal variables of equations will be coded with ‘0’.
z=0.683-2.694*(1)+1.666*(0)+0.821(0) -1.190* WBC (1)
z= -2.851Incorporating value of ‘z’ in equation 1
Probability of gangrenous cholecystitis=1/1+e(-2.851) =0.95Thus, the predictability model estimates 95% probability of gangrenous cholecystitis. Histopathological examination revealed gangrenous cholecystitis.
Patients with gangrenous cholecystitis usually present with non-specific and indistinguishable clinical and laboratory characteristics which make it difficult to differentiate these patients from those with acute cholecystitis. For those patients, we developed the second quick-and-easy model which was based on the assessment of two radiological variables such as GB wall thickening and pericholecystic fluid collection. Thus, a standard predicting model is for symptomatic patients and a quick-and-easy predicting model is for asymptomatic patients of gangrenous cholecystitis.
For an example, a 30-year-old female presented with 5 days history of non-specific abdominal pain. Her haemogram was normal. Her abdominal CT scan showed changes of acute cholecystitis with thickening GB wall and pericholecystic fluid collection. Here we first calculate ‘z’ in equation 3
z= -0.249-2.444* (1)+1.766* (1)
z= -2.693Incorporating value of ‘z’ in equation 1
Probability of gangrenous cholecystitis=1/1+e(-2.693) =0.94The estimated probability of gangrenous cholecystitis is 94%. The histopathological examination confirmed gangrenous cholecystitis.
The present predictive models have several advantages. First, our predictive models are based on clinical and radiological variables which can be easily obtained from laboratory examinations and CT findings or sonography results. Second, models have high predictability for gangrenous cholecystitis, so that it is possible to prioritise patients based on the severity of the disease.
Limitation
The results are based on the retrospective study; therefore the proposed predictive model must be validated prospectively. For this reason, a prospective database has been established to validate the trade recorded in the series. As only surgically managed patients were included in this study, it is hard to tell if this predictive model could be valid for non-surgically managed patients.
Conclusion
We developed a standard and quick-and-easy model to predict gangrenous cholecystitis in symptomatic and asymptomatic patients. The standard predictive model has the specificity of 82.2% and a sensitivity of 82%. The quick-and-easy model has the specificity of 78% and a sensitivity of 78.2%. The clinical application of the models is expected to improve the pre-operative diagnosis of gangrenous cholecystitis. Moreover, clinical application of both the models may prove a useful tool in the clinical decision making for urgent surgical intervention based on the predicted probability of gangrenous cholecystitis.
DM: Diabetes mellitus; IHD: Ischemic heart disease
DM: Diabetes mellitus; IHD: Ischemic heart disease; WBC: White blood cells; AST: Aspartate transaminase; GB: Gallbladder
p≤0.05
CI: Confidence interval
GB: Gallbladder
aLogistic regression equation predicts the log odds, the coefficients (column B) represent the difference between the log odds, a log odds ratio
bThe antilog of the coefficients
p≤0.05
CI: Confidence interval
GB: gallbladder
aLogistic regression equation predicts the log odds, the coefficients (column B) represent the difference between the log odds, a log odds ratio
bThe antilog of the coefficients
p≤0.05
CI: Confidence interval