Diabetic Retinopathy (DR) is among the most common disease which leads to the cause of blindness. The abnormality in the anatomic and pathological structure has to be analysed for the prevention of retinal diseases. In this review, the Optic Disc (OD), blood vessels and exudates has been studied. We searched databases like Google scholar, IEEE, Elsevier, Springer and other relevant databases to find literature on segmentation of OD, blood vessels and exudates (Pathology) carried out by the researchers with various image processing algorithms with publicly available datasets such as DRIVE, STARE, DRION, DIARETDB0, DIARETDB1, MESSIDOR, etc. After the wide search, we got 32 articles on the retinal disease DR for review. The search revealed that, exclusively for the retinal disease DR, the detection of abnormality in OD and blood vessels alone never gives fruitful results. Therefore, for patients with the chronic diseases DR, the pathological sign exudates have to be analysed by the radiologists while diagnosing and the patients not falling in the DR group can be diagnosed only with OD and blood vessels. From the review, it was inferred that, the morphological operation based segmentation gains more importance due to its better accuracy rate.
Introduction
In the retinal fundus images, the most significant task is the diagnosis of the retinal diseases. Ophthalmologists need an automation system for diagnosis with reduced time. Early diagnosis and treatment of different retinal diseases is extremely essential to prevent it from getting worse or even leading to blindness. Soft Computing techniques with image processing algorithms take part in analysing and assisting Ophthalmologists towards disease diagnosis. In the human retina, the OD serves as the landmark in order to locate other fundus features like macula and blood vessels [1]. The three major anatomical structures in colour fundus photographs are OD, fovea and the blood vessels.
Even though several techniques are available, still a complete comparison lacks in this area. Most of the OD methods are based on the fact that due to the convergence of the blood vessels, it is the brightest part of an image. The exudates detection depends partially on the result of OD detection. Detection of blood vessels involves huge challenges as images present inadequate contrast, lightning variations, noise influence and anatomic variability. Automated blood vessels detection can be suitably helpful for pre-screening system and for other clinical purposes to diagnose hypertension and cardiovascular diseases [2].
DR is the symptom of diabetes in the retinal fundus images which leads to the cause of blindness. The anatomical feature of OD is of prime importance for diagnosis as a main step in developing automated screening of DR. The OD is placed 3-4 mm to the nasal side of the fovea where it is located in the centre part of the macula region in retina.
Moreover, effective segmentation of blood vessels will improve the diagnosis system during clinical examination. The leakage of blood from blood vessels leads to the formation of exudates which can be identified as the brightest spots in the fundus images. Giving due consideration to the increase in occurrence of diabetes, the abnormalities of fundus images may be analysed using the anatomical structures OD, blood vessel and the sign of abnormality exudates.
We searched Google scholar, IEEE, Elsevier, Springer and other relevant databases for literature on the segmentation of anatomical structures such as OD and blood vessels. Also, the search was extended towards the abnormality of DR patient’s i.e., the exudates was also carried out. The search was also been concentrated on the publicly available datasets such as DRIVE, STARE, DRION, DIARETDB0, DIARETDB1, MESSIDOR used by the researchers in diagnosing DR.
Literature Review
Literature review for the OD, blood vessel and exudates with respect to the different techniques used are represented as block diagram shown in [Table/Fig-1].
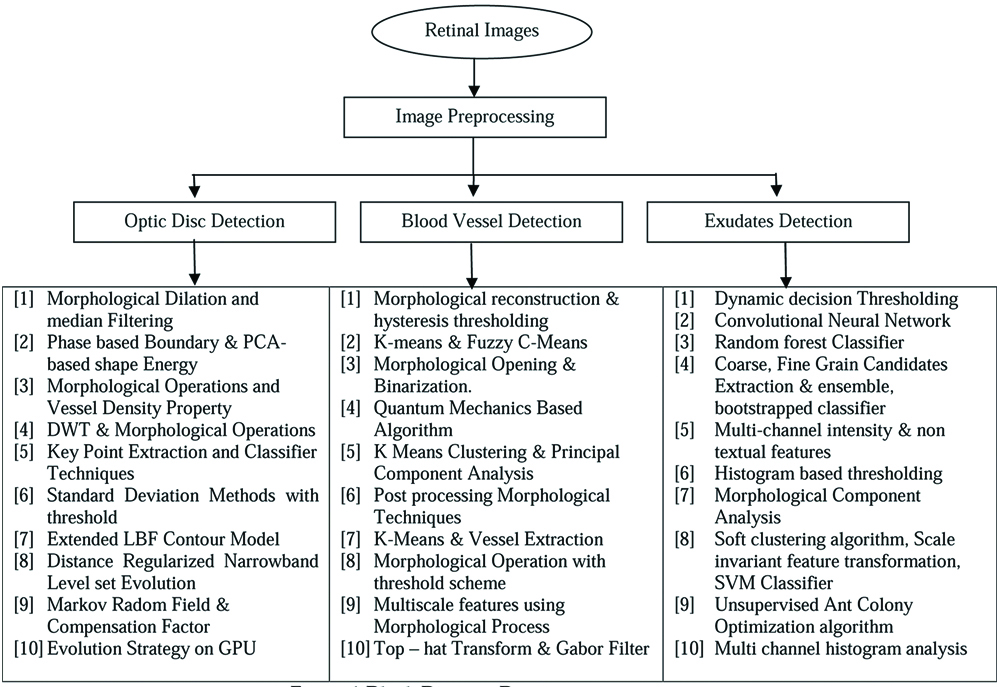
Preprocessing
The first preliminary step of image analysis is the preprocessing for automated retinal disease diagnosis. The preprocessing techniques involve denoising of an image, contrast enhancement and differentiation of foreground and background etc. Conversion of the RGB image into the Red, Green and Blue channel images in which features from each channel image are extracted for further processing [3].
Optic Disc Segmentation
OD is located using appropriate threshold and grayscale morphological dilation and median filtering for segmentation of the region. The proposed algorithm is tested on DRIVE, DIRATEDB0, DIRATEDB1 and DRIONS databases and the accuracy 100%, 96.92%, 98.98%, 100% is achieved [4]. The sensitivity and specificity is in the range 74.60-87.07%, 99.39-99.61%. In another work [5] author used phase-based boundary energy and PCA-based shape energy to identify the OD and these methods applied on the databases MESSIDOR, ONHSD and DRIONS. OD identification was done by enhancing the image using morphological operations and its region is detected automatically using vessel density property. The performance average values achieved for the proposed method is Jaccard coefficient (JCseg), Dice Coefficient (DCseg) and distance between OD centers are 0.8331, 0.9078 and 6.44, respectively [6]. Segmentation of OD based on Discrete Wavelet Transform (DWT) and morphological operations has been proposed [7]. The method was applied on the DIARETDB0, DIARETDB1 and obtained sensitivity and specificity of 71.54%, 99.53% and 71.11%, 99.56%. A research work [8], applied the keypoint extraction, texture analysis, visual dictionary, and classifier techniques on the database DIARETDB1, DRIVE, and ROC and obtain the results as an average of OD detection accuracy of 94.38%, 95.00%, and 90.00% is achieved, respectively.
Mean and standard deviation methods were used to detect optic nerve head region for applying threshold value and morphology operations for the segmentation of OD [9]. This algorithm was applied on the MESSIDOR database and the performance yielded is 92.06%. A novel implicit region based active Extended LBF contour model [10], was used to segment OD from the digital fundus images. The algorithm was applied on RIMONE and DIARETDB0 dataset and it gives results of an average F-score of 0.975, average boundary distance of 10.112 pixels and average correlation coefficient of 0.916. Distance Regularized Narrow Band Level Set Evolution (DRLSE) method for detecting OD has been used in a study [11], and the results attainted by the DRLSE method on DRIVE, DIARETDBI, MESSIDOR improves the sensitivity and specificity to 99.92% and 96.49% respectively. An automatic method called Atanassov’s Intuitionistic Fuzzy Set (A-IFS) was implemented to detect the OD [12], and the proposed algorithm applied on the DRIVE, MESSIDOR, DIARETDB0 dataset yielded an overall performance of 99% accuracy. The OD segmentation using two methods such as Markov Random Field (MRF) and Compensation Factor [13] was implemented. The first method segments the OD by removing vessels from OD region and the second method segments the OD using prior local intensity knowledge of the vessels. The author applies the proposed method on the DIARETDB1, DRIVE and STARE database. Evolution Strategy (ES) [14] was applied and implemented on GPU using CUDA (Compute Unified Device Architecture). The author performed these methods in coarse detection and contour edges refinement steps. The author implemented this method on DIARETDB and STARE and results obtained had 96% accuracy. The outline structure of the various researchers proposed with the anatomical feature OD detection has been shown as the graphical representation in [Table/Fig-2].
Graphical representation of OD detection.
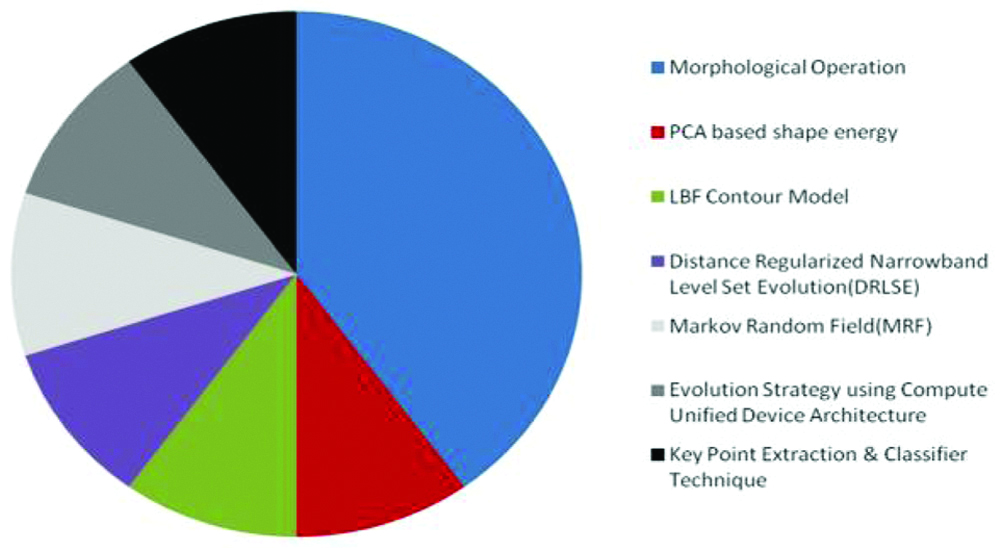
Blood Vessel Segmentation
The fundus image was initially preprocessed by enhancing the extracted green channel image using Contrast Limited Adaptive Histogram Equalization (CLAHE) and 2D Gabor wavelet. Morphological reconstruction and hysteresis thresholding methods were implemented in the enhanced image to obtain the blood vessels [15]. The method was implemented on the CHASE_DB1 and an accuracy of 0.950 was obtained. K-means and Fuzzy C-Means (FCM) were used by the researcher which also includes a hard and soft clustering stage, to get binary vessel map [16]. The post processing step was applied to remove false regions. Applying this clustering method achieved 95.94% and 95.71% accuracy for STARE and DRIVE datasets. Implementation of the basic preprocessing steps such as image acquisition, grayscale conversion and contrast enhancement, intensity adjustment, complement and adaptive histogram equalization was done and then the mathematical morphological opening, binarization and noise extraction methods were implemented on the preprocessed image and the specificity of 97%, a sensitivity of 69% and an accuracy of 94% occurred in the blood vessel detection [17]. Feature extraction methods such as 4-D Feature vector generation, optimized soft-max feature vector normalization and combined using optimized non-linear transformation were used for the detection of blood vessel. The proposed classification quantum mechanics-based algorithm is applied on the combined image to get the segmented blood vessel image [18]. This algorithm is tested on the database DRIVE and sensitivity, specificity, and accuracy obtained are 80.29%, 97.34%, and 95.83%, respectively.
A feature vector was formed by applying the preprocessed technique on the fundus images and then K-means clustering and Principal Component Analysis (PCA) is performed on the feature vector to group pixels as either vessel or non-vessel cluster [19]. The post processing morphological techniques implemented on the vessel segmented output from previous phase. The method was applied on the DRIVE database which yielded an accuracy of 95.36%. In another study [20], author used mathematical morphology methods such as Top-Hat Transform and Gaussian filtering methods for a preprocessing and K-Means and vessel extraction methods for classifying the blood vessels. The results obtained by the approach showed the effective average accuracy of 95.10% and best accuracy of 96.25%. Morphological operations for background elimination and noise removal algorithm were used for blood vessel enhancement [21]. Using the threshold scheme, vessel outline is then extracted. When applying the proposed method it gives an average vessel sensitivity of 70.87%, average specificity of 98% and average accuracy of 94.90% on STARE and DRIVE datasets. Multi-scale local statistical features based on gray level and morphology features combined with the features based on local area shape has been implemented to identify the blood vessel in pathological retinal image [22]. The accuracy of the proposed method is better than the 2nd human observer. Matched filtering and Hessian-based approach for vessel segmentation in standard fundus images was suggested for blood vessel segmentation [23]. This method was applied on HRF Dataset and an accuracy of 94% was revealed. The researcher [24], uses morphological top-hat transform and Gabor filter for the preprocessing and to obtain the binary vessel image the author proposed p-tile thresholding method. By applying this methods on STARE and DRIVE database, the author yields an accuracy of 94.02% and 94.59%, respectively. The morphological operations based segmentation of the blood vessels was used in [25] and the output are compared with matched filter using entropy thresholding. The method applied on the DRIVE database and the achieved average results sensitivity, specificity, accuracy is 78%, 22%, 84% on matched filter and 80%, 20%, 85% on morphological operations. [Table/Fig-3] represents the various researchers’ algorithms used for the blood vessel detection [15-24].
Algorithms used by the authors with respect to blood vessel detection [15-24].
Algorithms | Used by numbers of authors | Reference |
---|
Morphological Features and Operations | 06 | [15], [17], [20], [21], [22], [24] |
K-Means Algorithm | 03 | [16], [19], [20] |
Quantum Mechanics | 01 | [18] |
Hessian Based Approach | 01 | [23] |
Exudates Segmentation
Kaur J et al., applied dynamic decision thresholding irrespective of associated heterogeneity, bright and faint edges to segment exudates, applied on the STARE, MESSIDOR, DIARETDB1, e-Optha EX databases and the results are measured in terms of lesion level and segmentation for image-based evaluation. The sensitivity, specificity and accuracy of 88.85%, 96.15% and 93.46% for the lesion level and for the next 94.62%, 98.64% and 96.74% were achieved [26]. Tan JH et al., used convolutional neural network using 10 layers for detecting exudates automatically and simultaneously [27]. The proposed method applied on the CLEOPATRA database and achieved a sensitivity of 0.8758 and 0.7158 for exudates detection. Liu Q et al., used matched filters in the removal of an atomic structure, random forest classifier for classifying into exudate patches and exudate-free patches [28]. This method applies on the e-ophtha EX dataset and it achieved 76% in sensitivity and 75% in Positive Prediction Value (PPV). Fraz MM et al., implemented coarse level candidate extraction and Fine Grain Candidates Extraction methods as preprocessing methods to extract candidate exudates [29]. Then an ensemble classifier of bootstrapped decision trees used as a classifier. These methods are applied on the publically available dataset DIARETDB1, e-Ophtha EX, HEI-MED and MESSIDOR and the accuracy achieved is 0.8772, 0.8925, 0.9577, and 0.9836 for each of the dataset respectively.
Zhou W et al., segmented an entire image into a series of super pixels considered as candidates [30]. Including 19 multi-channel intensity features and novel contextual feature, total 20 features applied on the dataset DIARETDB1, and e-Ophtha EX, the results shows the advantages and effectiveness than other. Win KY et al., implements an automatic algorithm which uses histogram based thresholding information for detection of exudates on the DRIVE, DIARECTDB1, and 325 retinal fundus images from local dataset and achieves accurate rate of 99%, 90%, 89% [31]. Imani E et al., applies Morphological Component Analysis (MCA) as a first stage and dynamic thresholding and mathematical morphology technique is used for detection of exudates and gives result as AUC of 0.961 and 0.948 and 0.937 on DIARETDB1, HEI-MED and e-Ophtha datasets [32]. Ramasubramanian B et al., uses second order Gaussian filter for preprocessing, soft clustering algorithm for partitioned images into segments, Scale Invariant Feature Transformation (SIFT) algorithm and Support Vector Machine Classifier for training [33]. The proposed method applied on the 1000 image datasets and sensitivity of 99.96% and specificity of 96.60% was obtained. Pereira C et al., applied a new unsupervised ant colony optimization algorithm on the publically available datasets and the results obtained are better than the traditional Kirsch filter exudates detection algorithm [34]. Sharath Kumar PN et al., detected exudates using histogram analysis and eliminated false positives using multi-channel histogram analysis [35]. When the proposed method applied on the normal and abnormal images sensitivity and specificity 88.45% and 95.5% was obtained.
Discussion
Analysing the retinal disease has now changed with respect to the perspective of the radiologist and therefore, automatic detection precisely gains importance significantly in the medical field. A sizable assortment of writing has risen, utilizing utility-based overview techniques, on the significance of visual sharpness to a patient’s general prosperity. These examinations provide noteworthy outcomes with respect to the main significant structures which forms the base for the retinal diseases. It also gives a confirmation base to use in assessing the significance of new innovations to prevent or re-establish visual dedication. Also, the different database utilization has been narrated for the ease of the future researchers to proceed with the scope of work in the retinal image processing field with MATLAB software as shown in [Table/Fig-4].
Datasets used by the authors with respect to OD, blood vessel and exudates.
S.no | Database | Database used by number of authors |
---|
Optic disc | Blood vessel | Exudate |
---|
1 | DRIVE | 05 | 05 | 01 |
2 | DIAERCTDB0 | 03 | - | - |
3 | DIAERCTDB1 | 04 | - | 02 |
4 | STARE | 02 | 03 | 01 |
5 | MESSIDOR | 02 | - | - |
6 | ROC, RIMONE, DRIONS | 01 | - | - |
7 | HRF | - | 01 | - |
8 | CHASE_DB1 | - | 01 | - |
9 | E-Optha | - | - | 05 |
10 | HEI_MED | - | - | 02 |
11 | CLEORATRA | - | - | 01 |
Conclusion
From the review, it can be understood that, the morphological operation based segmentation of anatomical and pathological structure gives better accuracy rate than other techniques. This technique extends hands for the scholars to develop their research further in the scope of field.
[1]. Youssif AAA, Ghalwash AZ, Ghoneim AASA, Optic disc detection from normalized digital fundus images by means of a vessels direction matched filter IEEE Trans MedImag 2008 27(1):11-18.10.1109/TMI.2007.90032618270057 [Google Scholar] [CrossRef] [PubMed]
[2]. Honale SS, Kapse SV, A review of methods for blood vessel segmentation in retinal images International Journal of Engineering Research and Technology 2012 1(10):01-06. [Google Scholar]
[3]. Rasta SH, Partovi ME, Seyedarabi H, Javadzadeh A, A comparative study on preprocessing techniques in diabetic retinopathy retinal images: illumination correction and contrast enhancement J Med Signals and Sensors 2015 5(1):40-48. [Google Scholar]
[4]. Bharkad S, Automatic segmentation of optic disk in retinal images Biomedical Signal Processing and Control 2017 31:483-98.10.1016/j.bspc.2016.09.009 [Google Scholar] [CrossRef]
[5]. Dai B, Wu X, Bu W, Optic disc segmentation based on variational model with multiple energies Pattern Recognition 2017 64:226-35.10.1016/j.patcog.2016.11.017 [Google Scholar] [CrossRef]
[6]. Irshad S, Yin X, Li LQ, Salman U, Automatic optic disk segmentation in presence of disk blurring International Symposium on Visual Computing ISVC Advances in Visual Computing 2016 :13-23.10.1007/978-3-319-50835-1_2 [Google Scholar] [CrossRef]
[7]. Bharkad SD, Automatic Segmentation of Optic Disk in Retinal Images Using DWT. IEEE 6th International Conference on Advanced Computing 2016 :01-10.https://ieeexplore.ieee.org/document/754486710.1109/IACC.2016.78 [Google Scholar] [CrossRef]
[8]. Akyol K, Sen B, Bayır S, Automatic detection of optic disc in retinal image by using keypoint detection, texture analysis, and visual dictionary techniques Computational and Mathematical Methods in Medicine 2016 2016:681479110.1155/2016/681479127110272 [Google Scholar] [CrossRef] [PubMed]
[9]. Issac A, Parthasarthi M, Dutta MK, An Adaptive Threshold Based Algorithm for Optic Disc and Cup Segmentation in Fundus Images. IEEE 2nd International Conference on Signal Processing and Integrated Networks (SPIN) 2015 :143-47.https://ieeexplore.ieee.org/abstract/document/709538410.1109/SPIN.2015.7095384 [Google Scholar] [CrossRef]
[10]. Mittapalli PS, Kande GB, Segmentation of optic disc and optic cup from digital fundus images for the assessment of glaucoma Elsevier Biomedical Signal Processing and Control 2015 :34-46.10.1016/j.bspc.2015.09.003 [Google Scholar] [CrossRef]
[11]. Yu T, Ma Y, Li W, Automatic localization and segmentation of optic disc in fundus image using morphology and level set. IEEE 9th International Symposium on Medical information and Communication technology 2015 :195-99.https://ieeexplore.ieee.org/document/710752710.1109/ISMICT.2015.7107527 [Google Scholar] [CrossRef]
[12]. Karkuzhali S, Manimegalai D, Santhi D, Effective segmentation of optic disc in retinal images for diagnosing eye diseases that leads to blindness Biomedical & Pharmacology Journal 2015 8(1):427-34.10.13005/bpj/631 [Google Scholar] [CrossRef]
[13]. Gonzalez AS, Kaba D, Li Y, Liu X, Segmentation of blood vessels and optic disc in retinal images IEEE J Biomed Health Inform 2014 18(6):1874-86.10.1109/JBHI.2014.230274925265617 [Google Scholar] [CrossRef] [PubMed]
[14]. Torres GS, Taborda JA, Optic disk detection and segmentation of retinal images using an evolution strategy on GPU XVIII Symposium of Image, Signal Processing and Artificial Vision (STSIVA) 2013 :01-06.https://ieeexplore.ieee.org/document/664490410.1109/STSIVA.2013.6644904 [Google Scholar] [CrossRef]
[15]. Dash J, Bhoi N, A method for blood vessel segmentation in retinal images using morphological reconstruction IEEE International Conference on Computer, Electrical & Communication Engineering 2016 :01-08.https://ieeexplore.ieee.org/document/800956410.1109/ICCECE.2016.8009564 [Google Scholar] [CrossRef]
[16]. Yavuz Z, Köse C, Blood vessel extraction in colour retinal fundus images with enhancement filtering and unsupervised classification Journal of Healthcare Engineering 2017 2017:489725810.1155/2017/489725829065611 [Google Scholar] [CrossRef] [PubMed]
[17]. Bantan MT, Auto-segmentation of retinal blood vessels using image processing 4th Saudi International Conference on Information Technology (Big Data Analysis) (KACSTIT) 2016 :01-05.https://ieeexplore.ieee.org/document/775606810.1109/KACSTIT.2016.7756068 [Google Scholar] [CrossRef]
[18]. Youssry A, El-Rafei A, Elramly S, A quantum mechanics-based algorithm for vessel segmentation in retinal images Quantum Information Processing 2016 15(6):2303-23.10.1007/s11128-016-1292-1 [Google Scholar] [CrossRef]
[19]. Geetharamani R, Balasubramanian L, Retinal blood vessel segmentation employing image processing and data mining techniques for computerized retinal image analysis Biocybernetics and Biomedical Engineering 2016 36(1):102-18.10.1016/j.bbe.2015.06.004 [Google Scholar] [CrossRef]
[20]. Hassan G, El-Bendary N, Hassanien AE, Fahmy A, Abullah M, Shoeb , Retinal blood vessel segmentation approach based on mathematical morphology Procedia Computer Science 2015 65:612-22.10.1016/j.procs.2015.09.005 [Google Scholar] [CrossRef]
[21]. Singh D, Dharmveer , Singh B, A New Morphology based Approach for Blood Vessel Segmentation in Retinal Images Annual IEEE India Conference (INDICON) 2014 :01-06.https://ieeexplore.ieee.org/document/703068610.1109/INDICON.2014.7030686 [Google Scholar] [CrossRef]
[22]. Han Z, Yin Y, Meng X, Yang G, Yan X, Blood vessel segmentation in pathological retinal image IEEE International Conference on Data Mining Workshop 2014 :960-67.https://ieeexplore.ieee.org/document/702270010.1109/ICDMW.2014.16 [Google Scholar] [CrossRef]
[23]. Odstrcilik J, Kolar R, Jan J, Tornow RP, Budai A, Blood Vessel Segmentation in Video-Sequences From the Human Retina IEEE International Conference on Imaging Systems and Techniques (IST) 2014 :01-05.https://ieeexplore.ieee.org/document/695845910.1109/IST.2014.6958459 [Google Scholar] [CrossRef]
[24]. Yavuz Z, Kose C, Blood vessel segmentation from retinal images based on enhencement methods 22nd Signal Processing and Communications Applications IEEE Conference (SIU) 2014 :907-10.https://ieeexplore.ieee.org/document/683037710.1109/SIU.2014.6830377 [Google Scholar] [CrossRef]
[25]. Maruthusivarani M, Ramakrishnan T, Santhi D, Muthukkutti K, Comparison of automatic blood vessel segmentation methods in retinal images International Conference on Emerging Trends in VLSI, Embedded System, Nano Electronics and Telecommunication System (ICEVENT) 2013 :01-04.https://ieeexplore.ieee.org/document/649656910.1109/ICEVENT.2013.6496569 [Google Scholar] [CrossRef]
[26]. Kaur J, Mittal D, A generalized method for the segmentation of exudates from pathological retinal fundus images Biocybernetics and Biomedical Engineering 2018 38(1):27-53.10.1016/j.bbe.2017.10.003 [Google Scholar] [CrossRef]
[27]. Tan JH, Fujita H, Sivaprasad S, Bhandary SV, Krishnaraod A, Chua KC, Automated segmentation of exudates, haemorrhages, microaneurysms using single convolutional neural network Information Sciences 2017 420:66-76.10.1016/j.ins.2017.08.050 [Google Scholar] [CrossRef]
[28]. Liu Q, Zou B, Chen J, Ke W, Yue K, Chen Z, A location-to-segmentation strategy for automatic exudate segmentation in colour retinal fundus images Computerized Medical Imaging and Graphics 2017 55:78-86.10.1016/j.compmedimag.2016.09.00127665058 [Google Scholar] [CrossRef] [PubMed]
[29]. Fraz MM, Jahangir W, Zahid S, Hamayun MM, Barman SA, Multiscale segmentation of exudates in retinal images using contextual cues and ensemble classification Biomedical Signal Processing and Control 2017 35:50-62.10.1016/j.bspc.2017.02.012 [Google Scholar] [CrossRef]
[30]. Zhou W, Wu C, Yi Y, Du W, Automatic detection of exudates in digital colour fundus images using superpixel multi-feature classification IEEE Access 2017 5:17077-88.https://ieeexplore.ieee.org/document/801512310.1109/ACCESS.2017.2740239 [Google Scholar] [CrossRef]
[31]. Win KY, Choomchuay S, Automated detection of exudates using histogram analysis for Digital Retinal Images International Symposium on Intelligent Signal Processing and Communication Systems (ISPACS) 2016 :01-07.https://ieeexplore.ieee.org/document/782476810.1109/ISPACS.2016.7824768 [Google Scholar] [CrossRef]
[32]. Imani E, Pourreza HR, A novel method for retinal exudate segmentation using signal separation algorithm Computer Methods and Programs in Biomedicine 2016 133:195-05.10.1016/j.cmpb.2016.05.01627393810 [Google Scholar] [CrossRef] [PubMed]
[33]. Ramasubramanian B, Arunmani G, Ravivarma P, Rajasekar E, A Novel Approach for Automated Detection of Exudates Using Retinal Image Processing IEEE ICCSP conference 2015 :139-43.https://ieeexplore.ieee.org/document/732273510.1109/ICCSP.2015.7322735 [Google Scholar] [CrossRef]
[34]. Pereira C, Gonçalves L, Ferreira M, Exudate segmentation in fundus images using an ant colony optimization approach Information Sciences 2015 296:14-24.10.1016/j.ins.2014.10.059 [Google Scholar] [CrossRef]
[35]. Sharathkumar PN, Rajeshkumar R, Sathar A, Sahasranamam V, Automatic detection of exudates in retinal images using histogram analysis IEEE Recent Advances in Intelligent Computational Systems (RAICS) 2013 :277-81.https://ieeexplore.ieee.org/document/674548710.1109/RAICS.2013.6745487 [Google Scholar] [CrossRef]